Introduction
Welcome to our comprehensive guide on Artificial Intelligence (AI) using Python! In this introduction, we will explore some of the core ideas, techniques, and algorithms that lay the groundwork for AI. From understanding search algorithms to dealing with uncertainty and optimization, AI encompasses a variety of strategies that enable computers to perform tasks often deemed intelligent.
What is Artificial Intelligence?
Artificial intelligence is a broad field encompassing various techniques that allow computers to perform tasks that usually require human intelligence. Examples include:
- Image Recognition: AI can identify and understand faces in pictures.
- Game Playing: Computers can outperform humans in strategic games like chess and tic-tac-toe.
- Natural Language Understanding: AI can comprehend and respond to human speech or text.
Understanding Search Problems
One of the fundamentals of AI is the concept of search, which is central to solving problems. When faced with a challenge, AI aims to find solutions by systematically exploring possible actions. Let's dive deeper into the mechanisms of search problems.
Defining Key Terms
Before we get started, it's essential to understand some terminology:
- Agent: An entity that perceives its environment and takes actions.
- State: A configuration of the agent within its environment at a given time.
- Initial State: The starting point for the search.
- Actions: The set of choices available to the agent at any given state.
- Transition Model: Describes the outcome of performing an action in a specific state.
- Goal Test: A criterion used to determine if a state is the final goal.
- Path Cost: A numerical value indicating the cost of achieving a particular path from one state to another.
The Search Algorithm Framework
In approaching a search problem, we can follow a structured algorithm incorporating these key concepts. The general approach involves:
- Starting with an initial state.
- Expanding the state to generate child nodes.
- Evaluating the child nodes by applying the goal test.
- If the goal is reached, return the path.
- If not, continue to expand nodes until a solution is found or the frontier is empty.
Types of Search Strategies
We will now cover some practical search algorithms that are fundamental to AI.
Depth-First Search (DFS)
This approach explores the deepest nodes in the search tree first. Its main advantage is lower memory usage since it stores only the path from the root to the leaf node currently being explored. However, DFS can lead to getting stuck in cycles if not handled properly.
Breadth-First Search (BFS)
Unlike DFS, BFS explores the shallowest nodes first. This guarantees the shortest path to the solution, but at the cost of higher memory usage, as it needs to store all child nodes at the current depth before moving on. BFS is practical for problems where the goal is guaranteed to be at a shallower depth.
Optimization Techniques
Once we implement a search algorithm, we must consider optimization and efficiency in how we tackle search problems. Two crucial techniques include:
- Alpha-Beta Pruning: This technique helps reduce the number of nodes evaluated in the minimax algorithm by cutting branches that do not need to be explored. This ties into our exploration of the Minimax algorithm and its efficiency.
- Depth-Limited Search: Instead of exploring all nodes, set a depth ceiling to limit search depth. An evaluation function estimates the utility of searched nodes beyond the depth limit.
Adversarial Search: The Minimax Algorithm
In games like tic-tac-toe, where opponents play against each other, we need a strategy to handle adversarial conditions. This is where the minimax algorithm comes in. It aims to minimize the possible loss in a worst-case scenario. Let's break down its components:
- Minimax alternates between maximizing and minimizing values, reflecting the strategies of competing agents.
- It evaluates all potential outcomes and assigns scores based on terminal states: 1 for a win for the maximizing player, -1 for a loss, and 0 for a tie.
- Players make optimal moves by predicting the opponent's decisions and choosing the path that maximizes their score while minimizing potential losses.
Implementing Minimax
To implement Minimax effectively, we formulate recursive functions for both maximizing and minimizing values.
- MaxPlayer: Searches for the outcome with the highest value by evaluating all prospective states and selecting the one that maximizes their score.
- MinPlayer: Searches for the outcome with the lowest value, progressively narrowing down the board states by considering the opponent's potential moves.
Alpha-Beta Pruning in Minimax
With alpha-beta pruning, we can optimize Minimax by ignoring branches of the tree that cannot possibly affect the final outcome, thus speeding up the search. During the evaluation process, if the current state's score is less optimal than an already calculated outcome, it allows us to cut off exploration of that branch early.
Conclusion
In conclusion, search algorithms form the backbone of many artificial intelligence applications. From DFS, BFS to the Minimax Algorithm with optimizations like alpha-beta pruning, understanding these concepts is vital for building intelligent systems.
As we continue our journey into AI, the next steps involve looking into knowledge representation, how AI systems maintain and reason about the information they acquire. For further reading on AI foundations, consider checking out the article on Understanding Introduction to Deep Learning: Foundations, Techniques, and Applications or explore Understanding Generative AI: Concepts, Models, and Applications. Thank you for joining this exploration, and we look forward to seeing you in the next session!
[MUSIC] BRIAN YU: All right. Welcome, everyone, to an Introduction to Artificial Intelligence with Python.
My name is Brian Yu. And in this class, we'll explore some of the ideas, and techniques, and algorithms that are at the foundation of artificial intelligence.
Now, artificial intelligence covers a wide variety of types of techniques. Anytime you see a computer do something that appears to be intelligent or rational in some way,
like recognizing someone's face in a photo, or being able to play a game better than people can, or being able to understand human language when we talk to our phones
and they understand what we mean and are able to respond back to us, these are all examples of AI, or artificial intelligence. And in this class we'll explore some of the ideas that make that AI possible.
So we'll begin our conversations with search. The problem of, we have an AI and we would like the AI to be able to search for solutions to some kind of problem,
no matter what that problem might be. Whether it's trying to get driving directions from point A to point B, or trying to figure out how to play a game,
giving a tic-tac-toe game, for example, figuring out what move it ought to make. After that, we'll take a look at knowledge.
Ideally, we want our AI to be able to know information, to be able to represent that information, and more importantly, to be able to draw inferences from that information.
To be able to use the information it knows and draw additional conclusions. So we'll talk about how AI can be programmed in order to do just that. Then we'll explore the topic of uncertainty.
Talking about ideas of, what happens if a computer isn't sure about a fact but maybe is only sure with a certain probability? So we'll talk about some of the ideas behind probability
and how computers can begin to deal with uncertain events in order to be a little bit more intelligent in that sense, as well. After that, we'll turn our attention to optimization.
Problems of when the computer is trying to optimize for some sort of goal, especially in a situation where there might be multiple ways that a computer might solve a problem,
but we're looking for a better way or, potentially, the best way if that's at all possible. Then we'll take a look at machine learning, or learning more generally.
In looking at how when we have access to data our computers can be programmed to be quite intelligent by learning from data and learning from experience, being able to perform a task better and better
based on greater access to data. So your email, for example, where your email inbox somehow knows which of your emails are good emails and whichever emails are spam.
These are all examples of computers being able to learn from past experiences and past data. We'll take a look, too, at how computers are able to draw inspiration
from human intelligence, looking at the structure of the human brain and how neural networks can be a computer analog to that sort of idea. And how, by taking advantage of a certain type of structure of a computer
program, we can write neural networks that are able to perform tasks very, very effectively. And then finally, we'll turn our attention to language.
Not programming languages, but human languages that we speak every day. And taking a look at the challenges that come about as a computer tries to understand natural language
and how it is some of the natural language processing that occurs in modern artificial intelligence can actually work.
But today it will begin our conversation with search. This problem of trying to figure out what to do when we have some sort of situation that the computer is in,
some sort of environment that an agent is in, so to speak. And we would like for that agent to be able to somehow look for a solution to that problem.
Now, these problems can come in any number of different types of formats. One example, for instance, might be something like this classic 15 puzzle with the sliding tiles that you might
have seen, where you're trying to slide the tiles in order to make sure that all the numbers line up in order. This is an example of what you might call a search problem.
The 15 puzzle begins in an initially mixed up state and we need some way of finding moves to make in order to return the puzzle to its solved state.
But there are similar problems that you can frame in other ways. Trying to find your way through a maze, for example, is another example of a search problem.
You begin in one place, you have some goal of where you're trying to get to, and you need to figure out the correct sequence of actions that will take you from that initial state to the goal.
And while this is a little bit abstract, anytime we talk about maze solving in this class, you can translate it to something a little more real world,
something like driving directions. If you ever wonder how Google Maps is able to figure out what is the best way for you to get from point A to point B
and what turns to make, at what time, depending on traffic, for example. It's often some sort of search algorithm. You have an AI that is trying to get from an initial position
to some sort of goal by taking some sequence of actions. So we'll start our conversations today by thinking about these types of search problems and what
goes in to solving a search problem like this in order for an AI to be able to find a good solution. In order to do so, though, we're going to need
to introduce a little bit of terminology, some of which I've already used. But the first time we'll need to think about is an agent.
An agent is just some entity that perceives its environment, it somehow is able to perceive the things around it, and act on that environment in some way.
So in the case of the driving directions, your agent might be some representation of a car that is trying to figure out what actions to take in order
to arrive at a destination. In the case of the 15 puzzle with the sliding tiles, the agent might be the AI or the person that is trying to solve that puzzle,
trying to figure out what tiles to move in order to get to that solution. Next, we introduce the idea of a state. A state is just some configuration of the agent in its environment.
So in the 15 puzzle, for example, any state might be any one of these three for example. A state is just some configuration of the tiles.
Each of these states is different and is going to require a slightly different solution. A different sequence of actions will be needed in each one of these in order
to get from this initial state to the goal, which is where we're trying to get. The initial state then.
What is that? The initial state is just the state where the agent begins. It is one such state where we're going to start from
and this is going to be the starting point for our search algorithm, so to speak. We're going to begin with this initial state
and then start to reason about it, to think about what actions might we apply to that initial state in order to figure out how to get from the beginning to the end, from the initial position to whatever our goal happens to be.
And how do we make our way from that initial position to the goal? Well ultimately, it's via taking actions. Actions are just choices that we can make in any given state.
And in AI, we're always going to try to formalize these ideas a little bit more precisely such that we could program them a little bit more mathematically, so to speak.
So this will be a recurring theme and we can more precisely define actions as a function. We're going to effectively define a function called actions
that takes an input S, where S is going to be some state that exists inside of our environment, and actions of S is going to take the state as input and return as output the set of all actions that
can be executed in that state. And so it's possible that some actions are only valid in certain states and not in other states.
And we'll see examples of that soon, too. So in the case of the 15 puzzle, for example, they're generally going to be four possible actions
that we can do most of the time. We can slide a tile to the right, slide a tile to the left, slide a tile up, or slide a tile down, for example.
And those are going to be the actions that are available to us. So somehow our AI, our program, needs some encoding of the state, which is often going to be in some numerical format,
and some encoding of these actions. But it also needs some encoding of the relationship between these things, how do the states and actions relate to one another?
And in order to do that, we'll introduce to our AI a transition model, which will be a description of what state we get after we perform some available action in some other state.
And again, we can be a little bit more precise about this, define this transition model a little bit more formally, again, as a function.
The function is going to be a function called result, that this time takes two inputs. Input number one is S, some state.
And input number two is A, some action. And the output of this function result is it is going to give us the state that we get after we perform action A in state
S. So let's take a look at an example to see more precisely what this actually means. Here's an example of a state of the 15 puzzle, for example.
And here's an example of an action, sliding a tile to the right. What happens if we pass these as inputs to the result function? Again, the result function takes this board, this state, as its first input.
And it takes an action as a second input. And of course, here, I'm describing things visually so that you can see visually what the state is and what the action is.
In a computer, you might represent one of these actions as just some number that represents the action. Or if you're familiar with enums that allow
you to enumerate multiple possibilities, it might be something like that. And the state might just be represented as an array, or two dimensional array, of all of these numbers that exist.
But here we're going to show it visually just so you can see it. When we take this state and this action, pass it into the result function, the output is a new state.
The state we get after we take a tile and slide it to the right, and this is the state we get as a result. If we had a different action and a different state, for example,
and passed that into the result function, we'd get a different answer altogether. So the result function needs to take care
of figuring out how to take a state and take an action and get what results. And this is going to be our transition model that describes how it is that states and actions are related to each other.
If we take this transition model and think about it more generally and across the entire problem, we can form what we might call a state space, the set of all of the states we can get from the initial state
via any sequence of actions, by taking zero or one or two or more actions in addition to that, so we could draw a diagram that looks something like this.
Where every state is represented here by a game board. And there are arrows that connect every state to every other state we can get two from that state.
And the state space is much larger than what you see just here. This is just a sample of what the state space might actually look like. And, in general, across many search problems,
whether they're this particular 15 puzzle or driving directions or something else, the state space is going to look something like this. We have individual states and arrows that are connecting them.
And oftentimes, just for simplicity, we'll simplify our representation of this entire thing as a graph, some sequence of nodes and edges that connect nodes.
But you can think of this more abstract representation as the exact same idea. Each of these little circles, or nodes, is going to represent one of the states inside of our problem.
And the arrows here represent the actions that we can take in any particular state, taking us from one particular state to another state, for example.
All right. So now we have this idea of nodes that are representing these states, actions that can take us from one state to another,
and a transition model that defines what happens after we take a particular action. So the next step we need to figure out is
how we know when the AI is done solving the problem. The AI I needs some way to know when it gets to the goal, that it's found the goal.
So the next thing we'll need to encode into our artificial intelligence is a goal test, some way to determine whether a given state is a goal state. In the case of something like driving directions, it might be pretty easy.
If you're in a state that corresponds to whatever the user typed in as their intended destination, well, then you know you're in a goal state.
In the 15 puzzle, it might be checking the numbers to make sure they're all in ascending order. But the AI need some way to encode whether or not
any state they happen to be in is a goal. And some problems might have one goal, like a maze where you have one initial position and one ending position
and that's the goal. In other more complex problems, you might imagine that there are multiple possible goals, that there are multiple ways
to solve a problem. And we might not care which one the computer finds as long as it does find a particular goal.
However, sometimes a computer doesn't just care about finding a goal, but finding a goal well, or one with a low cost. And it's for that reason that the last piece
of terminology that we use to define these search problems is something called a path cost. You might imagine that in the case of driving directions,
it would be pretty annoying if I said I wanted directions from point A to point B, and the route the Google Maps gave me was a long route with lots of detours that were unnecessary, that took longer than it should
have for me to get to that destination. And it's for that reason that when we're formulating search problems, we'll often give every path some sort of numerical cost, some number telling us
how expensive it is to take this particular option. And then tell our AI that instead of just finding a solution, some way of getting from the initial state to the goal,
we'd really like to find one that minimizes this path cost, that is less expensive, or takes less time, or minimizes some other numerical value.
We can represent this graphically, if we take a look at this graph again. And imagine that each of these arrows, each of these actions that we can take from one state to another state,
has some sort of number associated with it, that number being the path cost of this particular action where some of the costs for any particular action
might be more expensive than the cost for some other action, for example. Although this will only happen in some sorts of problems. In other problems we can simplify the diagram
and just assume that the cost of any particular action is the same. And this is probably the case in something like the 15 puzzle, for example, where it doesn't really make a difference whether I'm
moving right or moving left. The only thing that matters is the total number of steps that I have to take to get from point A to point B. And each of those steps
is of equal cost. We can just assume it's a some constant cost, like one. And so this now forms the basis for what we
might consider to be a search problem. A search problem has some sort of initial state, some place where we begin, some sort of action that we can take
or multiple actions that we can take in any given state, and it has a transition model, some way of defining what happens when we go from one state and take one action,
what state do we end up with as a result. In addition to that, we need some goal test to know whether or not we've reached a goal.
And then we need a path cost function that tells us for any particular path, by following some sequence of actions, how expensive is that path. What is its cost in terms of money, or time,
or some other resource that we are trying to minimize our usage of. The goal, ultimately, is to find a solution, where a solution in this case is just some sequence of actions that will take us from the initial state
to the goal state. And, ideally, we'd like to find not just any solution, but the optimal solution, which is a solution that has the lowest path cost among all
of the possible solutions. And in some cases, there might be multiple optimal solutions, but an optimal solution just means that there
is no way that we could have done better in terms of finding that solution. So now we've defined the problem. And now we need to begin to figure out how
it is that we're going to solve this kind of search problem. And in order to do so, you'll probably imagine that our computer is going to need to represent a whole bunch of data
about this particular problem. We need to represent data about where we are in the problem. And we might need to be considering multiple different options at once.
And oftentimes when we're trying to package a whole bunch of data related to a state together, we'll do so using a data structure that we're going to call a node.
A node is a data structure that is just going to keep track of a variety of different values, and specifically in the case of a search problem,
it's going to keep track of these four values in particular. Every node is going to keep track of a state, the state we're currently on. And every node is also going to keep track of a parent.
A parent being the state before us, or the node that we used in order to get to this current state. And this is going to be relevant because eventually,
once we reach the goal node, once we get to the end, we want to know what sequence of actions we used in order to get to that goal. And the way we'll know that is by looking at these parents
to keep track of what led us to the goal, and what led us to that state, and what led us to the state before that, so on and so forth, backtracking our way to the beginning so that we
know the entire sequence of actions we needed in order to get from the beginning to the end. The node is also going to keep track of what action we took in order to get
from the parent to the current state. And the node is also going to keep track of a path cost. In other words, it's going to keep track of the number that
represents how long it took to get from the initial state to the state that we currently happen to be at. And we'll see why this is relevant as we start to talk about some
of the optimizations that we can make in terms of these search problems more generally. So this is the data structure that we're going
to use in order to solve the problem. And now let's talk about the approach, how might we actually begin to solve the problem?
Well, as you might imagine, what we're going to do is we're going to start at one particular state and we're just going to explore from there.
The intuition is that from a given state, we have multiple options that we could take, and we're going to explore those options.
And once we explore those options, we'll find that more options than that are going to make themselves available. And we're going to consider all of the available options
to be stored inside of a single data structure that we'll call the frontier. The frontier is going to represent all of the things that we could explore next, that we haven't yet explored or visited.
So in our approach, we're going to begin this search algorithm by starting with a frontier that just contains one state. The frontier is going to contain the initial state because at the beginning,
that's the only state we know about. That is the only state that exists. And then our search algorithm is effectively going to follow a loop.
We're going to repeat some process again and again and again. The first thing we're going to do is if the frontier is empty, then there's no solution.
And we can report that there is no way to get to the goal. And that's certainly possible. There are certain types of problems that an AI might
try to explore and realize that there is no way to solve that problem. And that's useful information for humans to know, as well. So if ever the frontier is empty, that means there's nothing left to explore,
and we haven't yet found a solution so there is no solution. There's nothing left to explore. Otherwise what we'll do is we'll remove a node from the frontier.
So right now at the beginning, the frontier just contains one node representing the initial state. But over time, the frontier might grow.
It might contain multiple states. And so here we're just going to remove a single node from that frontier. If that node happens to be a goal, then we found a solution.
So we remove a node from the frontier and ask ourselves, is this the goal? And we do that by applying the goal test that we talked about earlier, asking if we're at the destination or asking if all the numbers of the 15
puzzle happen to be in order. So if the node contains the goal, we found a solution. Great.
We're done. And otherwise, what we'll need to do is we'll need to expand the node. And this is a term in artificial intelligence.
To expand the node just means to look at all of the neighbors of that node. In other words, consider all of the possible actions that I could take from the state that this node as representing
and what nodes could I get to from there. We're going to take all of those nodes, the next nodes that I can get to from this current one I'm looking at,
and add those to the frontier. And then we'll repeat this process. So at a very high level, the idea is we start with a frontier that
contains the initial state. And we're constantly removing a node from the frontier, looking at where we can get to next, and adding those nodes to the frontier,
repeating this process over and over until either we remove a node from the frontier and it contains a goal, meaning we've solved the problem.
Or we run into a situation where the frontier is empty, at which point we're left with no solution. So let's actually try and take the pseudocode,
put it into practice by taking a look at an example of a sample search problem. So right here I have a sample graph. A is connected to B via this action, B is connected to node C and D, C
is connected to D, E is connected to F. And what I'd like to do is have my AI find a path from A to E. We want to get from this initial state to this goal state.
So how are we going to do that? Well, we're going to start with the frontier that contains the initial state.
This is going to represent our frontier. So our frontier, initially, will just contain A, that initial state where we're going to begin.
And now we'll repeat this process. If the frontier is empty, no solution. That's not a problem because the frontier is not empty.
So we'll remove a node from the frontier as the one to consider next. There is only one node in the frontier. So we'll go ahead and remove it from the frontier.
But now A, this initial node, this is the node we're currently considering. We follow the next step. We ask ourselves, is this node the goal?
No, it's not. A is not the goal. E is the goal.
So we don't return the solution. So instead, we go to this last step, expand the node and add the resulting nodes to the frontier.
What does that mean? Well, it means take this state A and consider where we could get to next. And after A what we could get to next is only
B. So that's what we get when we expand A. We find B. And we add B to the frontier. And now B is in the frontier and we repeat the process again.
We say, all right. The frontier is not empty. So let's remove B from the frontier.
B is now the node that we're considering. We ask ourselves, is B the goal? No, it's not.
So we go ahead and expand B and add its resulting nodes to the frontier. What happens when we expand B? In other words, what nodes can we get to from B?
Well, we can get to C and D. So we'll go ahead and add C and D from the frontier. And now we have two nodes in the frontier, C and D.
And we repeat the process again. We remove a node from the frontier, for now we'll do so arbitrarily just by picking C.
We'll see why later how choosing which node you remove from the frontier is actually quite an important part of the algorithm. But for now I'll arbitrarily remove C, say it's not the goal,
so we'll add E, the next one to the frontier. Then let's say I remove E from the frontier. And now I'm currently looking at state E. Is that a goal state?
It is because I'm trying to find a path from A to E. So I would return the goal. And that, now, would be the solution, that I'm now
able to return the solution and I found a path from A to E. So this is the general idea, the general approach of this search algorithm, to follow these steps constantly removing nodes from the frontier
until we're able to find a solution. So the next question you might reasonably ask is, what could go wrong here?
What are the potential problems with an approach like this? And here's one example of a problem that could arise from this sort of approach. Imagine this same graph, same as before, with one change.
The change being, now, instead of just an arrow from A to B, we also have an arrow from B to A, meaning we can go in both directions. And this is true in something like the 15 puzzle
where when I slide a tile to the right, I could then slide a tile to the left to get back to the original position. I could go back and forth between A and B.
And that's what these double arrows symbolize, the idea that from one state I can get to another and then I can get back. And that's true in many search problems.
What's going to happen if I try to apply the same approach now? Well, I'll begin with A, same as before. And I'll remove A from the frontier.
And then I'll consider where I can get to from A. And after A, the only place I can get choice B so B goes into the frontier. Then I'll say, all right.
Let's take a look at B. That's the only thing left in the frontier. Where can I get to from B? Before it was just C and D, but now because of that reverse arrow,
I can get to A or C or D. So all three A, C, and D. All of those now go into the frontier. They are places I can get to from B. And now I remove one from the frontier,
and, you know, maybe I'm unlucky and maybe I pick A. And now I'm looking at A again. And I consider where can I get to from A. And from A, well I can get to B.
And now we start to see the problem, that if I'm not careful, I go from A to B and then back to A and then to B again. And I could be going in this infinite loop where I never
make any progress because I'm constantly just going back and forth between two states that I've already seen. So what is the solution to this?
We need some way to deal with this problem. And the way that we can deal with this problem is by somehow keeping track of what we've already explored.
And the logic is going to be, well, if we've already explored the state, there's no reason to go back to it. Once we've explored a state, don't go back to it,
don't bother adding it to the frontier. There's no need to. So here is going to be our revised approach,
a better way to approach this sort of search problem. And it's going to look very similar just with a couple of modifications. We'll start with a frontier that contains the initial state.
Same as before. But now we'll start with another data structure, which would just be a set of nodes that we've already explored.
So what are the states we've explored? Initially, it's empty. We have an empty explored set.
And now we repeat. If the frontier is empty, no solution. Same as before.
We remove a node from the frontier, we check to see if it's a goal state, return the solution. None of this is any different so far.
But now, what we're going to do is we're going to add the node to the explored state. So if it happens to be the case that we remove a node from the frontier
and it's not the goal, we'll add it to the explored set so that we know we've already explored it. We don't need to go back to it again if it happens to come up later.
And then the final step, we expand the node and we add the resulting nodes to the frontier. But before we just always added the resulting nodes to the frontier,
we're going to be a little cleverer about it this time. We're only going to add the nodes to the frontier if they aren't already in the frontier and if they
aren't already in the explored set. So we'll check both the frontier and the explored set, make sure that the node isn't already in one of those two,
and so long as it isn't, then we'll go ahead and add to the frontier but not otherwise. And so that revised approach is ultimately
what's going to help make sure that we don't go back and forth between two nodes. Now the one point that I've kind of glossed over here
so far is this step here, removing a node from the frontier. Before I just chose arbitrarily, like let's just remove a node and that's it. But it turns out it's actually quite important
how we decide to structure our frontier, how we add them, and how we remove our nodes. The frontier is a data structure.
And we need to make a choice about in what order are we going to be removing elements? And one of the simplest data structures for adding and removing elements
is something called a stack. And a stack is a data structure that is a last in, first out data type. Which means the last thing that I add to the frontier
is going to be the first thing that I remove from the frontier. So the most recent thing to go into the stack, or the frontier in this case, is going to be the node that I explore.
So let's see what happens if I apply this stack based approach to something like this problem, finding a path from A to E. What's going to happen?
Well, again we'll start with A. And we'll say, all right. Let's go ahead and look at A first. And then, notice this time, we've added A to the explored set.
A is something we've now explored, we have this data structure that's keeping track. We then say from A we can get to B. And all right.
From B what can we do? Well from B, we can explore B and get to both C and D. So we added C and then D. So now when we explore a node,
we're going to treat the frontier as a stack, last in, first out. D was the last one to come in so we'll go ahead and explore that next. And say, all right, where can we get to from D?
Well we can get to F. And so, all right. We'll put F into the frontier. And now because the frontier is a stack, F
is the most recent thing that's gone in the stack. So F is what we'll explore next. We'll explore F and say, all right.
Where can we get you from F? Well, we can't get anywhere so nothing gets added to the frontier. So now what was the new most recent thing added to the frontier?
Well it's not C, the only thing left in the frontier. We'll explore that from which we can say, all right, from C we can get to E. So E goes into the frontier.
And then we say, all right. Let's look at E and E is now the solution. And now we've solved the problem.
So when we treat the frontier like a stack, a last in, first out data structure, that's the result we get. We go from A to B to D to F, and then we sort of backed up and went down
to C and then E. And it's important to get a visual sense for how this algorithm is working. We went very deep in this search tree, so
to speak, all the way until the bottom where we hit a dead end. And then we effectively backed up and explored this other route that we didn't try before.
And it's this going very deep in the search tree idea, this way the algorithm ends up working when we use a stack, that we call this version of the algorithm depth first search.
Depth first search is the search algorithm where we always explore the deepest node in the frontier. We keep going deeper and deeper through our search tree.
And then if we hit a dead end, we back up and we try something else instead. But depth first search is just one of the possible search options that we could use.
It turns out that there is another algorithm called breadth first search, which behaves very similarly to depth first search with one difference.
Instead of always exploring the deepest node in the search tree the way the depth first search does, breadth first search is always going to explore the shallowest node in the frontier.
So what does that mean? Well, it means that instead of using a stack, which depth first search, or DFS, used where the most recent item added
to the frontier is the one we'll explore next, in breadth first search, or BFS, will instead use a queue where a queue is a first in, first out data type, where the very first thing we add to the frontier is the first one
we'll explore. And they effectively form a line or a queue, where the earlier you arrive in the frontier, the earlier you get explored.
So what would that mean for the same exact problem finding a path from A to E? Well we start with A, same as before.
Then we'll go ahead and have explored A, and say, where can we get to from A? Well, from A we can get to B. Same as before. From B, same as before.
We can get to C and D so C and D get added to the frontier. This time, though, we added C to the frontier before D so we'll explore C first.
So C gets explored. And from C, where can we get to? Well, we can get to E.
So E gets added to the frontier. But because D was explored before E, we'll look at D next. So we'll explore D and say, where can we get to from D?
We can get to F. And only then will we say, all right. Now we can get to E. And so what breadth first search, or BFS, did is we started here, we looked at both C and D,
and then we looked at E. Effectively we're looking at things one away from the initial state, then two away from the initial state.
And only then, things that are three away from the initial state. Unlike depth first search, which just went as deep as possible into the search tree until it hit a dead end and then,
ultimately, had to back up. So these now are two different search algorithms that we could apply in order to try and solve a problem.
And let's take a look at how these would actually work in practice with something like maze solving, for example. So here's an example of a maze.
These empty cells represent places where our agent can move. These darkened gray cells and represent walls that the agent can't pass through.
And, ultimately, our agent, our AI, is going to try to find a way to get from position A to position B via some sequence of actions, where those actions are left,
right, up, and down. What will depth first search do in this case? Well depth first search will just follow one path.
If it reaches a fork in the road where it has multiple different options, depth first search is just, in this case, going to choose one. There isn't a real preference.
But it's going to keep following one until it hits a dead end. And when it hits a dead end, depth first search effectively goes back to the last decision point
and tries the other path. Fully exhausting this entire path and when it realizes that, OK, the goal is not here,
then it turns its attention to this path. It goes as deep as possible. When it hits a dead end, it backs up and then tries this other path,
keeps going as deep as possible down one particular path, and when it realizes that that's a dead end, then it'll back up. And then ultimately find its way to the goal.
And maybe you got lucky and maybe you made a different choice earlier on, but ultimately this is how depth first search is going to work. It's going to keep following until it hits a dead end.
And when it hits a dead end, it backs up and looks for a different solution. And so one thing you might reasonably ask is, is this algorithm always going to work?
Will it always actually find a way to get from the initial state to the goal? And it turns out that as long as our maze is finite, as long as they're that finitely many spaces where
we can travel, then yes. Depth first search is going to find a solution because eventually it will just explore everything.
If the maze happens to be infinite and there's an infinite state space, which does exist in certain types of problems, then it's a slightly different story. But as long as our maze has finitely many squares,
we're going to find a solution. The next question, though, that we want to ask is, is it going to be a good solution?
Is it the optimal solution that we can find? And the answer there is not necessarily. And let's take a look at an example of that.
In this maze, for example, we're again trying to find our way from A to B. And you notice here there are multiple possible solutions. We could go this way, or we could go up in order
to make our way from A to B. Now if we're lucky, depth first search will choose this way and get to B. But there's no reason, necessarily, why depth first search would choose
between going up or going to the right. It's sort of an arbitrary decision point because both are going to be added to the frontier.
And ultimately, if we get unlucky, depth first search might choose to explore this path first because it's just a random choice at this point.
It will explore, explore, explore, and it'll eventually find the goal, this particular path, when in actuality there was a better path.
There was a more optimal solution that used fewer steps, assuming we're measuring the cost of a solution based on the number of steps that we need to take.
So depth first search, if we're unlucky, might end up not finding the best solution when a better solution is available. So if that's DFS, depth first search.
How does BFS, or breadth first search, compare? How would it work in this particular situation? Well the algorithm is going to look very different visually
in terms of how BFS explores. Because BFS looks at shallower nodes first, the idea is going to be BFS will first look at all of the nodes that
are one away from the initial state. Look here and look here, for example. Just at the two nodes that are immediately next to this initial state.
Then it will explore nodes that are two away, looking at the state and that state, for example. Then it will explore nodes that are three away, this state and that state.
Whereas depth first search just picked one path and kept following it, breadth first search on the other hand, is taking the option of exploring all of the possible paths
kind of at the same time, bouncing back between them, looking deeper and deeper at each one, but making sure to explore the shallower ones or the ones that are
closer to the initial state earlier. So we'll keep following this pattern, looking at things that are four away, looking at things that are five away, looking
at things that are six away, until eventually we make our way to the goal. And in this case, it's true we had to explore some states that ultimately didn't lead us anywhere.
But the path that we found to the goal was the optimal path. This is the shortest way that we could get to the goal. And so, what might happen then in a larger maze?
Well let's take a look at something like this and how breadth first search is going to behave. Well, breadth first search, again, will just
keep following the states until it receives a decision point. It could go either left or right. And while DFS just picked one and kept following
that until it hit a dead end, BFS on the other hand, will explore both. It'll say, look at this node, then this node, and I'll look at this node, then that node, so on and so forth.
And when it hits a decision point here, rather than pick one left or two right and explore that path, it will again explore both alternating between them, going deeper and deeper.
Will explore here, and then maybe here and here, and then keep going. Explore here and slowly make our way, you can visually see further and further out.
Once we get to this decision point, we'll explore both up and down until, ultimately, we make our way to the goal.
And what you'll notice is, yes, breadth first search did find our way from A to B by following this particular path. But it needed to explore a lot of states in order to do so.
And so we see some trade here between DFS and BFS. That in DFS there may be some cases where there is some memory savings, as compared to a breadth first approach where
breadth first search, in this case, had to explore a lot of states. But maybe that won't always be the case. So now let's actually turn our attention to some code.
And look at the code that we could actually write in order to implement something like depth first search or breadth for the search in the context of solving a maze, for example.
So I'll go ahead and go into my terminal. And what I have here inside of maze.pi is an implementation of this same idea of maze solving.
I've defined a class called node that in this case is keeping track of the state, the parent, in other words the state before the state, and the action.
In this case, we're not keeping track of the path cost because we can calculate the cost of the path at the end after we found our way from the initial state to the goal.
In addition to this, I've defined a class called a stack frontier. And if unfamiliar with a class, a class is a way for me to define a way to generate objects in Python.
It refers to an idea of object oriented programming where the idea here is that I would like to create an object that is able to store all of my Frontier Data.
And I would like to have functions, otherwise known as methods on that object, that I can use to manipulate the object. And so what's going on here, if unfamiliar with the syntax,
is I have a function that initially creates a frontier that I'm going to represent using a list. And initially my frontier is represented by the empty list.
There's nothing in my frontier to begin with. I have an add function that adds something to the frontier, as by appending it to the end of the list.
I have a function that checks if the frontier contains a particular state. I have an empty function that checks if the frontier is empty. If the frontier is empty, that just means the length of the frontier
is zero. And then I have a function for removing something from the frontier. I can't remove something from the frontier if the frontier is empty.
So I check for that first. But otherwise, if the frontier isn't empty, recall that I'm implementing this frontier as a stack,
a last in, first out data structure. Which means the last thing I add to the frontier, in other words, the last thing in the list,
is the item that I should remove from this frontier. So what you'll see here is I have removed the last item of a list. And if you index into a Python list with negative one,
that gets you the last item in the list. Since zero is the first item, negative one kind of wraps around and gets you to the last item in the list.
So we give that the node. We call that node, we update the frontier here on line 28 to say, go ahead and remove that node that you just removed from the frontier.
And then we return the node as a result. So this class here effectively implements the idea of a frontier. It gives me a way to add something to a frontier and a way
to remove something from the frontier as a stack. I've also, just for good measure, implemented an alternative version of the same thing called a Q frontier.
Which, in parentheses you'll see here, it inherits from a stack frontier, meaning it's going to do all the same things that the stack frontier did, except the way we remove a node from the frontier
is going to be slightly different. Instead of removing from the end of the list the way we would in a stack, we're instead going to remove from the beginning of the list.
self.frontierzero will get me the first node in the frontier, the first one that was added. And that is going to be the one that we return in the case of a Q.
Under here I have a definition of a class called maze. This is going to handle the process of taking a sequence, a maze like text file, and figuring out how to solve it.
So we'll take as input a text file that looks something like this, for example, where we see hash marks that are here representing walls and I have the character A representing the starting position,
and the character B representing the ending position. And you can take a look at the code for parsing this text file right now. That's the less interesting part.
The more interesting part is this solve function here, where the solve function is going to figure out how to actually get from point A to point B.
And here we see an implementation of the exact same idea we saw from a moment ago. We're going to keep track of how many states
we've explored just so we can report that data later. But I start with a node that represents just the start state. And I start with a frontier that in this case is a stack frontier.
And given that I'm treating my frontier as a stack, you might imagine that the algorithm I'm using here is now depth first search. Because depth first search or DFS uses a stack as its data structure.
And initially, this frontier is just going to contain the start state. We initialize an explored set that initially is empty. There's nothing we've explored so far.
And now here's our loop, that notion of repeating something again and again. First, we check if the frontier is empty by calling that empty function that we saw the implementation of a moment ago.
And if the frontier is indeed empty, we'll go ahead and raise an exception, or a Python error, to say, sorry. There is no solution to this problem.
Otherwise, we'll go ahead and remove a node from the frontier, as by calling frontier.remove and update the number of states we've explored. Because now we've explored one additional state
so we say self.numexplored plus equals one, adding one to the number of states we've explored. Once we remove a node from the frontier, recall
that the next step is to see whether or not it's the goal, the goal test. And in the case of the maze, the goal is pretty easy. I check to see whether the state of the node is equal to the goal.
Initially when I set up the maze, I set up this value called goal which is the property of the maze so I can just check to see if the node is actually the goal.
And if it is the goal, then what I want to do is backtrack my way towards figuring out what actions I took in order to get to this goal.
And how do I do that? We'll recall that every node stores its parent-- the node that came before it that we used to get to this node--
and also the action used in order to get there. So I can create this loop where I'm constantly just looking at the parent of every node and keeping
track, for all of the parents, what action I took to get from the parent to this. So this loop is going to keep repeating this process of looking through all
of the parent nodes until we get back to the initial state, which has no parent, where node.parent is going to be equal to none. As I do so, I'm going to be building up the list of all
of the actions that I'm following and the list of all of the cells that are part of the solution. But I'll reverse them because when I build it
up going from the goal back to the initial state, I'm building the sequence of actions from the goal to the initial state, but I want to reverse them in order to get the sequence of actions
from the initial state to the goal. And that is, ultimately, going to be the solution. So all of that happens if the current state is equal to the goal.
And otherwise, if it's not the goal, well, then I'll go ahead and add this state to the explored set to say, I've explored this state now.
No need to go back to it if I come across it in the future. And then, this logic here implements the idea of adding neighbors to the frontier.
I'm saying, look at all of my neighbors. And I implemented a function called neighbors that you can take a look at. And for each of those neighbors, I'm going to check,
is the state already in the frontier? Is the state already in the explored set? And if it's not in either of those, then I'll go ahead and add this new child
node-- this new node-- to the frontier. So there's a fair amount of syntax here, but the key here
is not to understand all the nuances of the syntax, though feel free to take a closer look at this file on your own to get a sense for how it is working.
But the key is to see how this is an implementation of the same pseudocode, the same idea that we were describing a moment ago on the screen when we were looking at the steps that we might follow in order
to solve this kind of search problem. So now let's actually see this in action. I'll go ahead and run maze.py on maze1.txt, for example.
And what we'll see is here we have a printout of what the maze initially looked like. And then here, down below, is after we've solved it.
We had to explore 11 states in order to do it, and we found a path from A to B. And in this program, I just happened to generate a graphical representation of this, as well--
so I can open up maze.png, which is generated by this program-- that shows you where, in the darker color here, the wall is. Red is the initial state, green is the goal,
and yellow is the path that was followed. We found a path from the initial state to the goal. But now let's take a look at a more sophisticated maze
to see what might happen instead. Let's look now at maze2.txt, where now here we have a much larger maze. Again, we're trying to find our way from point A to point B,
but now you'll imagine that depth-first search might not be so lucky. It might not get the goal on the first try. It might have to follow one path then backtrack
and explore something else a little bit later. So let's try this. Run pythonmaze.py of maze2.txt, this time trying on this other maze.
And now depth-first search is able to find a solution. Here, as indicated by the stars, is a way to get from A to B. And we can represent this visually by opening up this maze.
Here's what that maze looks like. And highlighted in yellow, is the path that was found from the initial state to the goal.
But how many states do we have to explore before we found that path? Well, recall that, in my program, I was keeping track of the number of states that we've explored so far.
And so I can go back to the terminal and see that, all right, in order to solve this problem, we had to explore 399 different states. And in fact, if I make one small modification to the program
and tell the program at the end when we output this image, I added an argument called "show explored". And if I set "show explored" equal to true
and rerun this program pythonmaze.py by running it on maze2, and then I open the maze, what you'll see here is, highlighted in red, are all of the states that had to be explored to get from the initial state
to the goal. Depth-First Search, or DFS, didn't find its way to the goal right away. It made a choice to first explore this direction.
And when it explored this direction, it had to follow every conceivable path, all the way to the very end, even this long and winding one,
in order to realize that, you know what, that's a dead end. And instead, the program needed to backtrack. After going this direction, it must have gone this direction.
It got lucky here by just not choosing this path. But it got unlucky here, exploring this direction, exploring a bunch of states that it didn't need to and then, likewise,
exploring all of this top part of the graph when it probably didn't need to do that either. So all in all, depth-first search here really
not performing optimally, or probably exploring more states than it needs to. It finds an optimal solution, the best path to the goal, but the number of states needed to explore in order to do so,
the number of steps I had to take, that was much higher. So let's compare. How would Breadth-First Search, or BFS, do on this exact same maze instead?
And in order to do so, it's a very easy change. The algorithm for DFS and BFS is identical with the exception of what data structure we use to represent the frontier.
That in DFS I used a stack frontier-- last in, first out-- whereas in BFS, I'm going to use a queue frontier-- first in,
first out, where the first thing I add to the frontier is the first thing that I remove. So I'll go back to the terminal, rerun this program on the same maze,
and now you'll see that the number of states we had to explore was only 77, as compared to almost 400 when we used depth-first search. And we can see exactly why.
We can see what happened if we open up maze.png now and take a look. Again, yellow highlight is the solution that breath-first search found, which, incidentally, is the same solution that depth-first search found.
They're both finding the best solution, but notice all the white unexplored cells. There was much fewer states that needed to be explored
in order to make our way to the goal because breadth-first search operates a little more shallowly. It's exploring things that are close to the initial state
without exploring things that are further away. So if the goal is not too far away, then breadth-first search can actually behave quite effectively on a maze that
looks a little something like this. Now, in this case, both BFS and DFS ended up finding the same solution, but that won't always be the case.
And in fact, let's take a look at one more example, for instance, maze3.txt. In maze3.txt, notice that here there are multiple ways that you could get from A to B.
It's a relatively small maze, but let's look at what happens. If I use-- and I'll go ahead and turn off "show explored" so we just see the solution.
If I use BFS, breadth-first search, to solve maze3.txt, well, then we find a solution. And if I open up the maze, here's the solution that we found.
It is the optimal one. With just four steps, we can get from the initial state to what the goal happens to be.
But what happens if we try to use, depth-first search, or DFS, instead? Well, again, I'll go back up to my queue frontier, where queue frontier means that we're using breadth-first search.
And I'll change it to a stack frontier, which means that now we'll be using depth-first search. I'll rerun Pythonmaze.py.
And now you'll see that we find a solution, but it is not the optimal solution. This, instead, is what our algorithm finds.
And maybe depth-first search would have found this solution. It's possible, but it's not guaranteed, that if we just happen to be unlucky, if we choose this state instead of that state,
then depth-first search might find a longer route to get from the initial state to the goal. So we do see some trade-offs here where depth-first search might not
find the optimal solution. So at that point, it seems like breadth-first search is pretty good. Is that the best we can do, where it's going to find us the optimal solution
and we don't have to worry about situations where we might end up finding a longer path to the solution than what actually exists? Where the goal is far away from the initial state--
and we might have to take lots of steps in order to get from the initial state to the goal-- what ended up happening, is that this algorithm, BFS, ended up
exploring basically the entire graph, having to go through the entire maze in order to find its way from the initial state to the goal state. What we'd ultimately like is for our algorithm
to be a little bit more intelligent. And now what would it mean for our algorithm to be a little bit more intelligent, in this case?
Well, let's look back to where breadth-first search might have been able to make a different decision and consider human intuition in this process, as well.
Like, what might a human do when solving this maze that is different than what BFS ultimately chose to do? Well, the very first decision point that BFS made
was right here, when it made five steps and ended up in a position where it had a fork in the road. It could either go left or it could go right.
In these initial couple of steps, there was no choice. There was only one action that could be taken from each of those states. And so the search algorithm did the only thing
that any search algorithm could do, which is keep following that state after the next state. But this decision point is where things get a little bit interesting.
Depth-first search, that very first search algorithm we looked at, chose to say, let's pick one path and exhaust that path, see if anything that way has the goal, and if not, then let's
try the other way. Breadth-first search took the alternative approach of saying, you know what?
Let's explore things that are shallow, close to us first, look left and right, then back left and back right, so on and so forth, alternating between our options in the hopes of finding something nearby.
But ultimately, what might a human do if confronted with a situation like this of go left or go right? Well, a human might visually see that, all right,
I'm trying to get to state B, which is way up there, and going right just feels like it's closer to the goal. Like, it feels like going right should be
better than going left because I'm making progress towards getting to that goal. Now, of course, there are a couple of assumptions that I'm making here.
I'm making the assumption that we can represent this grid as, like, a two-dimensional grid, where I know the coordinates of everything.
I know that A is in coordinate 0,0, and B is in some other coordinate pair. And I know what coordinate I'm at now, so I can calculate that, yeah, going this way, that is closer to the goal.
And that might be a reasonable assumption for some types of search problems but maybe not in others. But for now, we'll go ahead and assume that--
that I know what my current coordinate pair and I know the coordinate x,y of the goal that I'm trying to get to. And in this situation, I'd like an algorithm that
is a little bit more intelligent and somehow knows that I should be making progress towards the goal, and this is probably the way to do that because, in a maze,
moving in the coordinate direction of the goal is usually, though not always, a good thing. And so here we draw a distinction between two different types of search
algorithms-- uninformed search and informed search. Uninformed search algorithms are algorithms like DFS and BFS, the two algorithms that we just looked at,
which are search strategies that don't use any problem specific knowledge to be able to solve the problem. DFS and BFS didn't really care about the structure
of the maze or anything about the way that a maze is in order to solve the problem. They just look at the actions available and choose from those actions,
and it doesn't matter whether if it's a maze or some other problem. The solution, or the way that it tries to solve the problem, is really fundamentally going to be the same.
What we're going to take a look at now is an improvement upon uninformed search. We're going to take a look at informed search.
Informed search are going to be search strategies that use knowledge specific to the problem to be able to better find a solution. And in the case of a maze, this problem specific knowledge
is something like, if I'm going to square that is geographically closer to the goal, that is better than being in a square that is geographically further away.
And this is something we can only know by thinking about this problem and reasoning about what knowledge might be helpful for our AI agent to know a little something about.
There are a number of different types of informed search. Specifically, first, we're going to look at a particular type of search algorithm called greedy best-first search.
Greedy Best-First Search, often abbreviated GBFS, is a search algorithm that, instead of expanding the deepest node, like DFS, or the shallowest node, like BFS,
this algorithm is always going to expand the node that it thinks is closest to the goal. Now, the search algorithm isn't going to know for sure whether it is the closest
thing to the goal, because if we knew what was closest to the goal all the time, then we would already have a solution. Like, the knowledge of what is close to the goal,
we could just follow those steps in order to get from the initial position to the solution. But if we don't know the solution-- meaning we don't know exactly
what's closest to the goal-- instead, we can use an estimate of what's closest to the goal, otherwise known as a heuristic--
just some way of estimating whether or not we're close to the goal. And we'll do so using a heuristic function, conventionally called h(n), that takes a state of input and returns our estimate of how close we
are to the goal. So what might this heuristic function actually look like in the case of a maze-solving algorithm?
Where we're trying to solve a maze, what does a heuristic look like? Well, the heuristic needs to answer a question, like between these two cells, C and D, which one is better?
Which one would I rather be in if I'm trying to find my way to the goal? Well, any human could probably look at this and tell you, you know what? D looks like it's better.
Even if the maze is a convoluted and you haven't thought about all the walls, D is probably better. And why is D better?
Well, because if you ignore the wall-- let's just pretend the walls don't exist for a moment and relax the problem, so to speak-- D, just in terms of coordinate pairs, is closer to this goal.
It's fewer steps that I would need to take to get to the goal, as compared to C, even if you ignore the walls. If you just know the x,y coordinate of C, and the x,y coordinate of the goal,
and likewise, you know the x,y coordinate of D, you can calculate that D, just geographically, ignoring the walls, looks like it's better.
And so this is the heuristic function that we're going to use, and it's something called the Manhattan distance, one specific type of heuristic, where the heuristic is, how many squares vertically
and horizontally and then left to right-- so not allowing myself to go diagonally, just either up or right or left or down. How many steps do I need to take to get from each of these cells to the goal?
Well, as it turns out, D is much closer. There are fewer steps. It only needs to take six steps in order to get to that goal.
Again here ignoring the walls. We've relaxed the problem a little bit. We're just concerned with, if you do the math,
subtract the x values from each other and the y values from each other, what is our estimate of how far we are away? We can estimate that D is closer to the goal than C is.
And so now we have an approach. We have a way of picking which node to remove from the frontier. And at each stage in our algorithm, we're
going to remove a node from the frontier. We're going to explore the node, if it has the smallest value for this heuristic function, if it has the smallest
Manhattan distance to the goal. And so what would this actually look like? Well, let me first label this graph, label this maze,
with a number representing the value of this heuristic function, the value of the Manhattan distance from any of these cells. So from this cell, for example, were one away from the goal.
From this cell, were two away from the goal. Three away, four away. Here we're five away, because we have to go one to the right and then four up.
From somewhere like here, the Manhattan distance is 2. We're only two squares away from the goal, geographically, even though in practices we're
going to have to take a longer path, but we don't know that yet. The heuristic is just some easy way to estimate how far we are away from the goal.
And maybe our heuristic is overly optimistic. It thinks that, yeah, we're only two steps away, when in practice, when you consider the walls, it might be more steps.
So the important thing here is that the heuristic isn't a guarantee of how many steps it's going to take. It is estimating.
It's an attempt at trying to approximate. And it does seem generally the case that the squares that look closer to the goal have smaller values for the heuristic function
than squares that are further away. So now, using greedy best-first search, what might this algorithm actually do? Well, again, for these first five steps, there's not much of a choice.
We started this initial state, A. And we say, all right. We have to explore these five states. But now we have a decision point.
Now we have a choice between going left and going right. And before, when DFS and BFS would just pick arbitrarily because it just depends on the order you throw these two nodes into the frontier--
and we didn't specify what order you put them into the frontier, only the order you take them out. Here we can look at 13 and 11 and say that, all right,
this square is a distance of 11 away from the goal, according to our heuristic, according to our estimate. And this one we estimate to be 13 away from the goal.
So between those two options, between these two choices, I'd rather have the 11. I'd rather be 11 steps away from the goal, so I'll go to the right.
We're able to make an informed decision because we know a little something more about this problem. So then we keep following 10, 9, 8--
between the two sevens. We don't really have much of a way to know between those. So then we do just have to make an arbitrary choice.
And you know what? Maybe we choose wrong. But that's OK because now we can still say, all right, let's try this seven.
We say seven, six. We have to make this choice even though it increases the value of the heuristic function.
But now we have another decision point between six and eight. And between those two-- and really, we're also considering the 13, but that's much higher.
Between six, eight, and 13, well, the six is the smallest value, so we'd rather take the six. We're able to make an informed decision that going this way to the right
is probably better than going that way. So we turn this way. We go to five.
And now we find a decision point where we'll actually make a decision that we might not want to make, but there's unfortunately not too much of a way around this.
We see four and six. Four looks closer to the goal, right? It's going up, and the goal is further up.
So we end up taking that route, which ultimately leads us to a dead end. But that's OK because we can still say, all right, now let's try the six, and now follow this route that will ultimately lead us to the goal.
And so this now is how greedy best-first search might try to approach this problem, by saying whenever we have a decision between multiple nodes that we could explore,
let's explore the node that has the smallest value of h(n), this heuristic function that is estimating how far I have to go. And it just so happens that, in this case,
we end up doing better, in terms of the number of states we needed to explore, than BFS needed to. BFS explored all of this section and all of that section.
But we were able to eliminate that by taking advantage of this heuristic, this knowledge about how close we are to the goal or some estimate of that idea.
So this seems much better. So wouldn't we always prefer an algorithm like this over an algorithm like breadth-first search?
Well, maybe. One thing to take into consideration is that we need to come up with a good heuristic.
How good the heuristic is is going to affect how good this algorithm is. And coming up with a good heuristic can oftentimes be challenging. But the other thing to consider is to ask
the question, just as we did with the prior two algorithms, is this algorithm optimal? Will it always find the shortest path from the initial state to the goal?
And to answer that question, let's take a look at this example for a moment. Take a look at this example. Again, we're trying to get from A to B, and again, I've
labeled each of the cells with their Manhattan distance from the goal, the number of squares up and to the right you would need to travel in order to get from that square to the goal.
And let's think about, would greedy best-first search that always picks the smallest number end up finding the optimal solution? What is the shortest solution, and would this algorithm find it?
And the important thing to realize is that right here is the decision point. We're estimate to be 12 away from the goal. And we have two choices.
We can go to the left, which we estimate to be 13 away from the goal, or we can go up, where we estimate it to be 11 away from the goal. And between those two, greedy best-first search is going to say,
the 11 looks better than the 13. And in doing so, greedy best-first search will end up finding this path to the goal.
But it turns out this path is not optimal. There is a way to get to the goal using fewer steps. And it's actually this way, this way that ultimately involved fewer steps,
even though it meant at this moment choosing the worst option between the two-- or what we estimated to be the worst option, based on the heretics.
And so this is what we mean by this is a greedy algorithm. It's making the best decision, locally. At this decision point, it looks like it's better
to go here than it is to go to the 13. But in the big picture, it's not necessarily optimal, that it might find a solution when in actuality there
was a better solution available. So we would like some way to solve this problem. We like the idea of this heuristic, of being
able to estimate the path, the distance between us and the goal, and that helps us to be able to make better decisions and to eliminate having to search through entire parts of the state
space. But we would like to modify the algorithm so that we can achieve optimality, so that it can be optimal.
And what is the way to do this? What is the intuition here? Well, let's take a look at this problem.
In this initial problem, greedy best-first search found this solution here, this long path. And the reason why it wasn't great is because, yes, the heuristic numbers
went down pretty low, but later on, and they started to build back up. They built back 8, 9, 10, 11-- all the way up to 12, in this case. And so how might we go about trying to improve this algorithm?
Well, one thing that we might realize is that, if we go all the way through this algorithm, through this path, and we end up going to the 12, and we've had to take this many steps-- like,
who knows how many steps that is-- just to get to this 12, we could have also, as an alternative, taken much fewer steps, just six steps, and ended up at this 13 here.
And yes, 13 is more than 12, so it looks like it's not as good, but it required far fewer steps. Right?
It only took six steps to get to this 13 versus many more steps to get to this 12. And while greedy best-first search says, oh, well, 12 is better than 13
so pick the 12, we might more intelligently say, I'd rather be somewhere that heuristically looks like it takes slightly longer if I can get there much more quickly.
And we're going to encode that idea, this general idea, into a more formal algorithm known as A star search. A star search is going to solve this problem by,
instead of just considering the heuristic, also considering how long it took us to get to any particular state. So the distinction is greedy best-first search, if I am in a state
right now, the only thing I care about is what is the estimated distance, the heuristic value, between me and the goal.
Whereas A star search will take into consideration two pieces of information. It'll take into consideration, how far do I estimate I am from the goal,
but also how far did I have to travel in order to get here? Because that is relevant, too. So we'll search algorithms by expanding the node with the lowest
value of g(n) plus h(n). h(n) is that same heuristic that we were talking about a moment ago that's going to vary based on the problem, but g(n) is going to be the cost to reach
the node-- how many steps I had to take, in this case, to get to my current position. So what does that search algorithm look like in practice?
Well, let's take a look. Again, we've got the same maze. And again, I've labeled them with their Manhattan distance.
This value is the h(n) value, the heuristic estimate of how far each of these squares is away from the goal. But now, as we begin to explore states, we
care not just about this heuristic value but also about g(n), the number of steps I had to take in order to get there. And I care about summing those two numbers together.
So what does that look like? On this very first step, I have taken one step. And now I am estimated to be 16 steps away from the goal.
So the total value here is 17. Then I take one more step. I've now taken two steps.
And I estimate myself to be 15 away from the goal-- again, a total value of 17. Now I've taken three steps.
And I'm estimated to be 14 away from the goal, so on and so forth. Four steps, an estimate of 13. Five steps, estimate of 12.
And now, here's a decision point. I could either be six steps away from the goal with a heuristic of 13 for a total of 19, or I could be six steps away
from the goal with a heuristic of 11 with an estimate of 17 for the total. So between 19 and 17, I'd rather take the 17-- the 6 plus 11.
So so far, no different than what we saw before. We're still taking this option because it appears to be better. And I keep taking this option because it appears to be better.
But it's right about here that things get a little bit different. Now I could be 15 steps away from the goal with an estimated distance of 6. So 15 plus 6, total value of 21.
Alternatively, I could be six steps away from the goal-- because this was five steps away, so this is six steps away-- with a total value of 13 as my estimate.
So 6 plus 13-- that's 19. So here we would evaluate g(n) plus h(n) to be 19--
6 plus 13-- whereas here, we would be 15 plus 6, or 21. And so the intuition is, 19 less than 21, pick here. But the idea is ultimately I'd rather be having taken fewer steps to get to a 13
than having taken 15 steps and be at a six because it means I've had to take more steps in order to get there. Maybe there's a better path this way.
So instead we'll explore this route. Now if we go one more-- this is seven steps plus 14, is 21, so between those two it's sort of a toss up.
We might end up exploring that one anyways. But after that, as these numbers start to get bigger in the heuristic values and these heuristic values start to get smaller,
you'll find that we'll actually keep exploring down this path. And you can do the math to see that at every decision point, A star search is going to make a choice based on the sum of how many steps
it took me to get to my current position and then how far I estimate I am from the goal. So while we did have to explore some of these states,
the ultimate solution we found was, in fact, an optimal solution. It did find us the quickest possible way to get from the initial state to the goal.
And it turns out that A* is an optimal search algorithm under certain conditions. So the conditions are h of n, my heuristic, needs to be admissible.
What does it mean for a heuristic to be admissible? Well, a heuristic is admissible if it never overestimates the true cost. Each event always needs to either get it exactly right
in terms of how far away I am, or it needs to underestimate. So we saw an example from before where the heuristic value was much smaller than the actual cost it would take.
That's totally fine. But the heuristic value should never overestimate. It should never think that I'm further away from the goal than I actually am.
And meanwhile, to make a stronger statement, h of n also needs to be consistent. And what does it mean for it to be consistent?
Mathematically, it means that for every node, which we'll call n, and successor, the node after me, that I'll call n prime, where it takes a cost of c to make that step, the heuristic value of n
needs to be less than or equal to the heuristic value of n prime plus the cost. So it's a lot of math, but in words, what it ultimately
means is that if I am here at this state right now, the heuristic value from me to the goal shouldn't be more than the heuristic value of my successor, the next place I could go to, plus however much
it would cost me to just make that step, from one step to the next step. And so this is just making sure that my heuristic is consistent between all of these steps that I might take.
So as long as this is true, then A* search is going to find me an optimal solution. And this is where much of the challenge of solving these search problems can
sometimes come in, that A* search is an algorithm that is known, and you could write the code fairly easily. But it's choosing the heuristic that can be the interesting challenge.
The better the heuristic is, the better I'll be able to solve the problem, and the fewer states that I'll have to explore. And I need to make sure that the heuristic satisfies
these particular constraints. So all in all, these are some of the examples of search algorithms that might work.
And certainly, there are many more than just this. A*, for example, does have a tendency to use quite a bit of memory, so there are alternative approaches to A* that ultimately use less memory than
this version of A* happens to use. And there are other search algorithms that are optimized for other cases as well.
But now, so far, we've only been looking at search algorithms where there's one agent. I am trying to find a solution to a problem.
I am trying to navigate my way through a maze. I am trying to solve a 15 puzzle. I am trying to find driving directions from point A to point B.
Sometimes in search situations, though, we'll enter an adversarial situation where I am an agent trying to make intelligent decisions,
and there is someone else who is fighting against me, so to speak, that has an opposite objective, someone where I am trying to succeed, someone else that wants me to fail.
And this is most popular in something like a game, a game like tic-tac-toe, where we've got this 3-by-3 grid, and X and O take turns either writing an X or an O in any one of these squares.
And the goal is to get three X's in a row, if you're the X player, or three O's in a row, if you're the O player. And computers have gotten quite good at playing games, tic-tac-toe very easily,
but even more complex games. And so you might imagine, what does an intelligent decision in a game look like?
So maybe X makes an initial move in the middle, and O plays up here. What does an intelligent move for X now become? Where should you move if you were X?
And it turns out there are a couple of possibilities. But if an AI is playing this game optimally, then the AI might play somewhere like the upper right, where
in this situation, O has the opposite objective of X. X is trying to win the game, to get three in a row diagonally here, and O is trying to stop that objective, opposite of the objective.
And so O is going to place here, to try to block. But now, X has a pretty clever move. X can make a move, like this where now X has two possible ways that X
can win the game. X could win the game by getting three in a row across here, or X could win the game by getting three in a row vertically this way.
So it doesn't matter where O makes their next move. O could play here, for example, blocking the three in a row horizontally, but then X is going to win the game by getting a three in a row vertically.
And so there's a fair amount of reasoning that's going on here in order for the computer to be able to solve a problem. And it's similar in spirit to the problems we've looked at so far.
There are actions, there's some sort of state of the board, and some transition from one action to the next, but it's different in the sense that this is now not just a classical search
problem, but an adversarial search problem, that I am the X player, trying to find the best moves to make, but I know that there is some adversary that is trying to stop me.
So we need some sort of algorithm to deal with these adversarial type of search situations. And the algorithm we're going to take a look at
is an algorithm called Minimax, which works very well for these deterministic games, where there are two players. It can work for other types of games as well, but we'll look right now at games
where I make a move, that my opponent makes a move, and I am trying to win, and my opponent is trying to win, also. Or in other words, my opponent is trying to get me to lose.
And so what do we need in order to make this algorithm work? Well, anytime we try and translate this human concept, of playing a game, winning, and losing, to a computer, we want
to translate it in terms that the computer can understand. And ultimately, the computer really just understands numbers. And so we want some way of translating a game of X's and O's
on a grid to something numerical, something the computer can understand. The computer doesn't normally understand notions of win or lose, but it does understand the concept of bigger and smaller.
And so what we might yet do is, we might take each of the possible ways that a tic-tac-toe game can unfold and assign a value, or a utility, to each one of those possible ways.
And in a tic-tac-toe game, and in many types of games, there are three possible outcomes. The outcomes are, O wins, X wins, or nobody wins.
So player one wins, player two wins, or nobody wins. And for now, let's go ahead and assign each of these possible outcomes a different value.
We'll say O winning-- that'll have a value of negative 1. Nobody winning-- that'll have a value of 0.
And X winning-- that will have a value of 1. So we've just assigned numbers to each of these three possible outcomes. And now, we have two players.
We have the X player and the O player. And we're going to go ahead and call the X player the max player. And we'll call the O player the min player.
And the reason why is because in the Minimax algorithm, the max player, which in this case is X, is aiming to maximize the score. These are the possible options for the score, negative 1, 0, and 1.
X wants to maximize the score, meaning if at all possible, X would like this situation where X wins the game. And we give it a score of 1.
But if this isn't possible, if X needs to choose between these two options, negative 1 meaning O winning, or 0 meaning nobody winning, X would rather that nobody wins, score of 0, than a score of negative 1,
O winning. So this notion of winning and losing in time has been reduced mathematically to just this idea of, try and maximize
the score. The X player always wants the score to be bigger. And on the flip side, the min player, in this case, O,
is aiming to minimize the score. The O player wants the score to be as small as possible. So now we've taken this game of X's and O's and winning and losing
and turned it into something mathematical, something where X is trying to maximize the score, O is trying to minimize the score. Let's now look at all of the parts of the game
that we need in order to encode it in an AI so that an AI can play a game like tic-tac-toe. So the game is going to need a couple of things.
We'll need some sort of initial state, that we'll in this case call S0, which is how the game begins, like an empty tic-tac-toe board, for example.
We'll also need a function called player, where the player function is going to take as input a state, here represented by S. And the output of the player function is going to be,
which player's turn is it? We need to be able to give a tic-tac-toe board to the computer, run it through a function, and that function tells us whose turn it is.
We'll need some notion of actions that we can take. We'll see examples of that in just a moment. We need some notion of a transition model-- same as before.
If I have a state, and I take an action, I need to know what results as a consequence of it. I need some way of knowing when the game is over.
So this is equivalent to kind of like a goal test, but I need some terminal test, some way to check to see if a state is a terminal state, where a terminal state means
the game is over. In the classic game of tic-tac-toe , a terminal state means either someone has gotten three in a row, or all of the squares of the tic-tac-toe board are
filled. Either of those conditions make it a terminal state. In a game of chess, it might be something like,
when there is checkmate, or if checkmate is no longer possible, that becomes a terminal state. And then finally we'll need a utility function, a function that takes a state
and gives us a numerical value for that terminal state, some way of saying, if X wins the game, that has a value of 1. If O has won the game, that has the value of negative 1.
If nobody has won the game, that has a value of 0. So let's take a look at each of these in turn. The initial state, we can just represent in tic-tac-toe as the empty game board.
This is where we begin. It's the place from which we begin this search. And again, I'll be representing these things visually.
But you can imagine this really just being an array, or a two-dimensional array, of all of these possible squares. Then we need the player function that, again, takes a state
and tells us whose turn it is. Assuming X makes the first move, if I have an empty game board, then my player function is going to return X
And if I have a game board where X has made a move, that my player function is going to return O. The player function takes a tic-tac-toe game board and tells us whose turn it is.
Next up, we'll consider the actions function. The actions function, much like it did in classical search, takes a state and gives us the set of all of the possible actions
we can take in that state. So let's imagine it's O's turn to move in a game board that looks like this. What happens when we pass it into the actions function?
So the actions function takes this state of the game as input, and the output is a set of possible actions it's a set of-- I could move in the upper left, or I could move in the bottom middle.
Those are the two possible action choices that I have when I begin in this particular state. Now, just as before, when we add states and actions,
we need some sort of transition model to tell us, when we take this action in the state, what is the new state that we get? And here, we define that using the result function that takes
a state as input, as well as an action. And when we apply the result function to this state, saying that let's let O move in this upper left corner, the new state we get
is this resulting state, where O is in the upper-left corner. And now, this seems obvious to someone who knows how to play tic-tac-toe. Of course, you play in the upper left corner--
that's the board you get. But all of this information needs to be encoded into the AI. The AI doesn't know how to play tic-tac-toe
until you tell the AI how the rules of tic-tac-toe work. And this function, defining the function here, allows us to tell the AI how this game actually works
and how actions actually affect the outcome of the game. So the AI needs to know how the game works. The AI also needs to know when the game is over.
That is by defining a function called terminal that takes as input a state S, such that if we take a game that is not yet over, pass it into the terminal function, the output is false.
The game is not over. But if we take a game that is over, because X has gotten three in a row along that diagonal, pass that into the terminal function,
then the output is going to be true, because the game now is, in fact, over. And finally, we've told the AI how the game works in terms of what moves can be made and what happens when you make those moves.
We've told the AI when the game is over. Now we need to tell the AI what the value of each of those states is. And we do that by defining this utility function, that takes a state, S,
and tells us the score or the utility of that state. So again, we said that if X wins the game, that utility is a value of 1, whereas if O wins the game, then the utility of that is negative 1.
And the AI needs to know, for each of these terminal states where the game is over, what is the utility of that state? So I can give you a game board like this, where the game is, in fact, over,
and I ask the AI to tell me what the value of that state is, it could do so. The value of the state is 1. Where things get interesting, though, is if the game is not yet over.
Let's imagine a game board like this. We're in the middle of the game. It's O's turn to make a move.
So how do we know it's O's turn to make a move? We can calculate that, using the player function. We can say, player of S, pass in the state.
O is the answer, so we know it's O's turn to move. And now, what is the value of this board, and what action should O take? Well that's going to depend.
We have to do some calculation here. And this is where the Minimax algorithm really comes in. Recall that X is trying to maximize the score, which means
that O is trying to minimize the score. O would like to minimize the total value that we get at the end of the game. And because this game isn't over yet, we don't really
know just yet what the value of this game board is. We have to do some calculation in order to figure that out. So how do we do that kind of calculation?
Well, in order to do so, we're going to consider, just as we might in a classical search situation, what actions could happen next, and what states will that take us to?
And it turns out that in this position, there are only two open squares, which means there are only two open places where O can make a move.
O could either make a move in the upper left, or O can make a move in the bottom middle. And Minimax doesn't know right out of the box which of those moves
is going to be better, so it's going to consider both. But now we run into the same situation. Now I have two more game boards, neither of which is over.
What happens next? And now it's in this sense that Minimax is what we'll call a recursive algorithm.
It's going to now repeat the exact same process, although now considering it from the opposite perspective. It's as if I am now going to put myself-- if I am the O player,
I'm going to put myself in my opponent's shoes, my opponent as the X player, and consider, what would my opponent do if they were in this position? What would my opponent do, the X player, if they were in that position?
And what would then happen? Well, the other player, my opponent, the X player, is trying to maximize the score, whereas I am trying
to minimize the score as the O player. So X is trying to find the maximum possible value that they can get. And so what's going to happen?
Well, from this board position, X only has one choice. X is going to play here, and they're going to get three in a row. And we know that that board, X winning--
that has a value of 1. If X wins the game, the value of that game board is 1. And so from this position, if this state can only ever lead to this state,
it's the only possible option, and this state has a value of 1, then the maximum possible value that the X player can get from this game board is also 1 from here.
The only place we can get is to a game with the value of 1, so this game board also has a value of 1. Now we consider this one over here.
What's going to happen now? Well, X needs to make a move. The only move X can make is in the upper left, so X will go there.
And in this game, no one wins the game. Nobody has three in a row. So the value of that game board is 0.
Nobody's won. And so again, by the same logic, if from this board position, the only place we can get to is a board where the value is 0,
then this state must also have a value of 0. And now here comes the choice part, the idea of trying to minimize. I, as the O player, now know that if I make this choice,
moving in the upper left, that is going to result in a game with a value of 1, assuming everyone plays optimally. And if I instead play in the lower middle,
choose this fork in the road, that is going to result in a game board with a value of 0. I have two options.
I have a 1 and a 0 to choose from, and I need to pick. And as the min player, I would rather choose the option with the minimum value.
So whenever a player has multiple choices, the min player will choose the option with the smallest value. The max player will choose the option with the largest value.
Between the 1 in the 0, the 0 is smaller, meaning I'd rather tie the game than lose the game. And so this game board, we'll say, also has a value of 0,
because if I am playing optimally, I will pick this fork in the road. I'll place my O here to block X's three in a row. X will move in the upper left, and the game will be over,
and no one will have won the game. So this is now the logic of Minimax, to consider all of the possible options that I can take, all of the actions that I can take,
and then to put myself in my opponent's shoes. I decide what move I'm going to make now by considering what move my opponent will make on the next turn.
And to do that, I consider what move I would make on the turn after that, so on and so forth, until I get all the way down to the end of the game, to one of these so-called terminal states.
In fact, this very decision point, where I am trying to decide as the O player what to make a decision about, might have just been a part of the logic that the X player, my opponent,
was using the move before me. This might be part of some larger tree where X is trying to make a move in this situation
and needs to pick between three different options in order to make a decision about what to happen. And the further and further away we are from the end of the game,
the deeper this tree has to go, because every level in this tree is going to correspond to one move, one move or action that I take, one move or action that my opponent takes, in order to decide what happens.
And in fact, it turns out that if I am the X player in this position, and I recursively do the logic and see I have a choice-- three choices, in fact, one of which leads to a value of 0, if I play here,
and if everyone plays optimally, the game will be a tie. If I play here, then O is going to win, and I'll lose, playing optimally. Or here, where I, the X player, can win--
well, between a score of 0 and negative 1 and 1, I'd rather pick the board with a value of 1, because that's the maximum value I can get.
And so this board would also have a maximum value of 1. And so this tree can get very, very deep, especially as the game starts to have more and more moves.
And this logic works not just for tic-tac-toe, but any of these sorts of games where I make a move, my opponent makes a move, and ultimately, we have these adversarial objectives.
And we can simplify the diagram into a diagram that looks like this. This is a more abstract version of the Minimax tree, where these are each states, but I'm no longer representing them as exactly
like tic-tac-toe boards. This is just representing some generic game that might be tic-tac-toe, might be some other game altogether.
Any of these green arrows that are pointing up-- that represents a maximizing state. I would like the score to be as big as possible.
And any of these red arrows pointing down-- those are minimizing states, where the player is the min player, and they are trying to make the score as small as possible.
So if you imagine in this situation, I am the maximizing player, this player here, and I have three choices-- one choice gives me a score of 5, one choice gives me a score of 3,
and one choice gives me a score of 9. Well, then, between those three choices, my best option is to choose this 9 over here, the score that maximizes my options out
of all the three options. And so I can give this state a value of 9, because among my three options, that is the best
choice that I have available to me. So that's my decision now. You imagine it's like one move away from the end of the game.
But then you could also ask a reasonable question. What might my opponent do two moves away from the end of the game? My opponent is the minimizing player.
They are trying to make the score as small as possible. Imagine what would have happened if they had to pick which choice to make. One choice leads us to this state, where I, the maximizing player,
am going to opt for 9, the biggest score that I can get. And one leads to this state, where I, the maximizing player, would choose 8, which is then the largest score than I can get.
Now, the minimizing player, forced to choose between a 9 or an 8, is going to choose the smallest possible score, which in this case is an 8. And that is, then, how this process would unfold.
But the minimizing player, in this case, considers both of their options, and then all of the options that would happen as a result of that.
So this now is a general picture of what the Minimax algorithm looks like. Let's now try to formalize it using a little bit of pseudocode. So what exactly is happening in the Minimax algorithm?
Well, given a state, S, we need to decide what to happen. The max player-- if it's the max player's turn, then max is going to pick an action, A, in actions of S. Recall
that actions is a function that takes a state and gives me back all of the possible actions that I can take. It tells me all of the moves that are possible.
The max player is going to specifically pick an action, A, in the set of actions that gives me the highest value of min value of result of S and A. So what does that mean?
Well, it means that I want to make the option that gives me the highest score of all of the actions, A. But what score is that going to have?
To calculate that, I need to know what my opponent, the min player, is going to do if they try to minimize the value of the state that results. So we say, what state results after I take this action,
and what happens when the min player tries to minimize the value of that state? I consider that for all of my possible options.
And after I've considered that for all of my possible options, I pick the action, A, that has the highest value. Likewise, the min player is going to do the same thing, but backwards.
They're also going to consider, what are all of the possible actions they can take if it's their turn? And they're going to pick the action, A, that has the smallest
possible value of all the options. And the way they know what the smallest possible value of all the options is, is by considering what the max player is going to do,
by saying, what's the result of applying this action to the current state, and then, what would the max player try to do? What value would the max player calculate for that particular state?
So everyone makes their decision based on trying to estimate what the other person would do. And now we need to turn our attention to these two
functions, maxValue and minValue. How do you actually calculate the value of a state if you're trying to maximize its value, and how do you
calculate the value of a state if you're trying to minimize the value? If you can do that, then we have an entire implementation of this Minimax algorithm.
So let's try it. Let's try and implement this maxValue function that takes a state and returns as output the value of that state
if I'm trying to maximize the value of the state. Well, the first thing I can check for is to see if the game is over, because if the game is over--
in other words, if the state is a terminal state-- then this is easy. I already have this utility function that tells me
what the value of the board is. If the game is over, I just check, did X win? Did O win?
Is that a tie? And the utility function just knows what the value of the state is. What's trickier is if the game isn't over,
because then I need to do this recursive reasoning about thinking, what is my opponent going to do on the next move? Then I want to calculate the value of this state,
and I want the value of the state to be as high as possible. And I'll keep track of that value in a variable called v. And if I want the value to be as high as possible,
I need to give v an initial value. And initially, I'll just go ahead and set it to be as low as possible, because I don't know what options are available to me yet.
So initially, I'll set v equal to negative infinity, which seems a little bit strange, but the idea here is, I want the value initially to be as low as possible,
because as I consider my actions, I'm always going to try and do better than v. And if I set v to negative infinity, I know I can always do better than that.
So now I consider my actions. And this is going to be some kind of loop, where for every action in actions of state--
recall, actions is a function that takes my state and gives me all the possible actions that I can use in that state. So for each one of those actions, I want to compare it to v and say,
all right, v is going to be equal to the maximum of v and this expression. So what is this expression? Well, first it is, get the result of taking the action and the state,
and then get the min value of that. In other words, let's say, I want to find out from that state what is the best that the min player can do,
because they are going to try and minimize the score. So whatever the resulting score is of the min value of that state, compare it to my current best value, and just pick the maximum of those two,
because I am trying to maximize the value. In short, what these three lines of code are doing are going through all of my possible actions and asking the question,
how do I maximize the score, given what my opponent is going to try to do? After this entire loop, I can just return v, and that is now the value of that particular state.
And for the min player, it's the exact opposite of this, the same logic, just backwards. To calculate the minimum value of a state,
first we check if it's a terminal state. If it is, we return its utility. Otherwise, we're going to now try to minimize the value of the state,
given all of my possible actions. So I need an initial value for v, the value of the state. And initially, I'll set it to infinity, because I know it can always
get something less than infinity. So by starting with v equals infinity, I make sure that the very first action I find--
that will be less than this value of v. And then I do the same thing-- loop over all of my possible actions, and for each
of the results that we could get when the max player makes their decision, let's take the minimum of that and the current value of v. So after all is said and done I get the smallest possible value of v,
that I then return back to the user. So that, in effect, is the pseudocode for Minimax. That is how we take a game and figure out what the best move to make
is by recursively using these maxValue and minValue functions, where maxValue calls minValue, minValue calls maxValue, back and forth, all the way until we reach a terminal state, at which point
our algorithm can simply return the utility of that particular state. What you might imagine is that this is going to start to be a long process, especially as games start
to get more complex, as we start to add more moves and more possible options and games that might last quite a bit longer. So the next question to ask is, what sort of optimizations can we make here?
How can we do better in order to use less space or take less time to be able to solve this kind of problem? And we'll take a look at a couple of possible optimizations.
But for one, we'll take a look at this example. Again, we're turning to these up arrows and down arrows. Let's imagine that I now am the max player, this green arrow.
I am trying to make the score as high as possible. And this is an easy game, where there are just two moves. I make a move, one of these three options,
and then my opponent makes a move, one of these three options, based on what move I make. And as a result, we get some value.
Let's look at the order in which I do these calculations and figure out if there are any optimizations I might be able to make to this calculation process.
I'm going to have to look at these states one at a time. So let's say I start here on the left and say, all right, now I'm going to consider, what will the min player, my opponent, try to do here?
Well, the min player is going to look at all three of their possible actions and look at their value, because these are terminal states. They're the end of the game.
And so they'll see, all right, this node is a value of 4, value of 8, value of 5. And the min player is going to say, well, all right.
Between these three options, 4, 8, and 5, I'll take the smallest one I'll take the 4. So this state now has a value of 4.
Then I as the max player say, all right, if I take this action, it will have a value of 4. That's the best that I can do, because min player
is going to try and minimize my score. So now, what if I take this option? We'll explore this next.
And now I explore what the min player would do if I choose this action. And the min player is going to say, all right, what are the three options? The min player has options between 9, 3, and 7, and so 3
is the smallest among 9, 3, and 7. So we'll go ahead and say this state has a value of 3. So now I, as the max player--
I have now explored two of my three options. I know that one of my options will guarantee me a score of 4, at least, and one of my options will guarantee me a score of 3.
And now I consider my third option and say, all right, what happens here? Same exact logic-- the min player is going to look at these three states, 2, 4, and 6,
say the minimum possible option is 2, so the min player wants the two. Now I, as the max player, have calculated all of the information by looking two layers deep, by looking at all of these nodes.
And I can now say, between the 4, the 3, and the 2, you know what? I'd rather take the 4, because if I choose this option, if my opponent plays optimally,
they will try and get me to the 4, but that's the best I can do. I can't guarantee a higher score, because if I pick either of these two options, I might get a 3, or I might get a 2.
And it's true that down here is a 9, and that's the highest score of any of the scores. So I might be tempted to say, you know what?
Maybe I should take this option, because I might get the 9. But if the min player is playing intelligently, if they're making the best moves at each possible option
they have when they get to make a choice, I'll be left with a 3, whereas I could better, playing optimally, have guaranteed that I would get the 4.
So that doesn't affect the logic that I would use as a Minimax player trying to maximize my score from that node there. But it turns out, that took quite a bit of computation
for me to figure that out. I had to reason through all of these nodes in order to draw this conclusion. And this is for a pretty simple game, where I have three choices,
my opponent has three choices, and then the game's over. So what I'd like to do is come up with some way to optimize this. Maybe I don't need to do all of this calculation to still reach
the conclusion that, you know what? This action to the left-- that's the best that I could do.
Let's go ahead and try again and try and be a little more intelligent about how I go about doing this. So first, I start the exact same way.
I don't know what to do initially, so I just have to consider one of the options and consider what the min player might do. Min has three options, 4, 8, and 5.
And between those three options, min says, 4 is the best they can do, because they want to try to minimize the score. Now, I, the max player, will consider my second option,
making this move here and considering what my opponent would do in response. What will the min player do? Well, the min player is going to, from that state, look at their options.
And I would say, all right. 9 is an option, 3 is an option. And if I am doing the math from this initial state,
doing all this calculation, when I see a 3, that should immediately be a red flag for me, because when I see a 3 down here at this state,
I know that the value of this state is going to be at most 3. It's going to be 3 or something less than 3, even though I haven't yet looked at this last action or even further actions
if there were more actions that could be taken here. How do I know that? Well, I know that the min player is going to try to minimize my score.
And if they see a 3, the only way this could be something other than a 3 is if this remaining thing that I haven't yet looked at is less than 3, which means there is no way for this value to be anything more than 3,
because the min player can already guarantee a 3, and they are trying to minimize my score. So what does that tell me?
Well, it tells me that if I choose this action, my score is going to be 3, or maybe even less than 3, if I'm unlucky. But I already know that this action will guarantee me a 4.
And so given that I know that this action guarantees me a score of 4, and this action means I can't do better than 3, if I'm trying to maximize my options, there
is no need for me to consider this triangle here. There is no value, no number that could go here, that would change my mind between these two options.
I'm always going to opt for this path that gets me a 4, as opposed to this path, where the best I can do is a 3, if my opponent plays optimally.
And this is going to be true for all of the future states that I look at, too. But if I look over here, at what min player might do over here, if I see that this state is a 2, I know that this state is at most a 2,
because the only way this value could be something other than 2 is if one of these remaining states is less than a 2, and so the min player would opt for that instead.
So even without looking at these remaining states, I, as the maximizing player, can know that choosing this path to the left is going to be better than choosing either of those two paths to the right,
because this one can't be better than 3, this one can't be better than 2, and so 4 in this case is the best that I can do. And I can say now that this state has a value of 4.
So in order to do this type of calculation, I was doing a little bit more bookkeeping, keeping track of things, keeping track all the time of, what is the best that I can do,
what is the worst that I can do, and for each of these states, saying, all right, well, if I already know that I can get a 4, then if the best I can do at this state is a 3,
no reason for me to consider it. I can effectively prune this leaf and anything below it from the tree. And it's for that reason this approach, this optimization to Minimax,
is called alpha-beta pruning. Alpha and beta stand for these two values that you'll have to keep track of, the best you can do so far
and the worst you can do so far. And pruning is the idea of, if I have a big, long, deep search tree, I might be able to search it more efficiently if I don't
need to search through everything, if I can remove some of the nodes to try and optimize the way that I look through this entire search space. So alpha-beta pruning can definitely save us a lot of time
as we go about the search process by making our searches more efficient. But even then, it's still not great as games get more complex. Tic-tac-toe, fortunately, is a relatively simple game,
and we might reasonably ask a question like, how many total possible tic-tac-toe games are there? You can think about it.
You can try and estimate, how many moves are there at any given point? How many moves long can the game last? It turns out there are about 255,000 possible tic-tac-toe games that
can be played. But compare that to a more complex game, something like a game of chess, for example--
far more pieces, far more moves, games that last much longer. How many total possible chess games could there be? It turns out that after just four moves each,
four moves by the white player, four moves by the black player, that there are 288 billion possible chess games that can result from that situation, after just four moves each.
And going even further. If you look at entire chess games and how many possible chess games there could be as a result there, there are more than 10
to the 29,000 possible chess games, far more chess games than could ever be considered. And this is a pretty big problem for the Minimax algorithm, because the Minimax
algorithm starts with an initial state, considers all the possible actions and all the possible actions after that, all the way until we get to the end of the game.
And that's going to be a problem if the computer is going to need to look through this many states, which is far more than any computer could ever do in any reasonable amount of time.
So what do we do in order to solve this problem? Instead of looking through all these states, which is totally intractable for a computer, we need some better approach.
And it turns out that better approach generally takes the form of something called depth-limited Minimax. Where normally Minimax is depth-unlimited--
we just keep going, layer after layer, move after move, until we get to the end of the game-- depth-limited Minimax is instead going to say, you know what?
After a certain number of moves-- maybe I'll look 10 moves ahead, maybe I'll look 12 moves ahead, but after that point, I'm going to stop and not consider additional moves that
might come after that, just because it would be computationally intractable to consider all of those possible options. But what do we do after we get 10 or 12 moves deep,
and we arrive at a situation where the game's not over? Minimax still needs a way to assign a score to that game board or game state to figure out what its current value is, which
is easy to do if the game is over, but not so easy to do if the game is not yet over. So in order to do that, we need to add one additional feature
to depth-limited Minimax called an evaluation function, which is just some function that is going to estimate the expected utility of a game from a given state.
So in a game like chess, if you imagine that a game value of 1 means white wins, negative 1 means black wins, 0 means it's a draw, then you might imagine that a score of 0.8 means white is very likely to win,
though certainly not guaranteed. And you would have an evaluation function that estimates how good the game state happens to be.
And depending on how good that evaluation function is, that is ultimately what's going to constrain how good the AI is. The better the AI is at estimating how good
or how bad any particular game state is, the better the AI is going to be able to play that game. If the evaluation function is worse and not as good
as estimating what the expected utility is, then it's going to be a whole lot harder. And you can imagine trying to come up with these evaluation functions.
In chess, for example, you might write an evaluation function based on how many pieces you have, as compared to how many pieces your opponent has, because each one
has a value in your evaluation function. It probably needs to be a little bit more complicated than that to consider other possible situations that
might arise as well. And there are many other variants on Minimax that add additional features in order to help it perform better
under these larger and more computationally intractable situations, where we couldn't possibly explore all of the possible moves, so we need to figure out how to use evaluation
functions and other techniques to be able to play these games, ultimately, better. But this now was a look at this kind of adversarial search, these search
problems where we have situations where I am trying to play against some sort of opponent. And these search problems show up all over the place
throughout artificial intelligence. We've been talking a lot today about more classical search problems, like trying to find directions from one location to another.
But anytime an AI is faced with trying to make a decision like, what do I do now in order to do something that is rational, or do something that is intelligent, or trying to play a game,
like figuring out what move to make, these sort of algorithms can really come in handy. It turns out that for tic-tac-toe, the solution is pretty simple,
because it's a small game. XKCD has famously put together a webcomic where he will tell you exactly what move to make as the optimal move
to make, no matter what your opponent happens to do. This type of thing is not quite as possible for a much larger game like checkers or chess,
for example, where chess is totally computationally intractable for most computers to be able to explore all the possible states.
So we really need our AI to be far more intelligent about how they go about trying to deal with these problems and how they go about taking this environment
that they find themselves in and ultimately searching for one of these solutions. So this, then, was a look at search and artificial intelligence.
Next time we'll take a look at knowledge, thinking about how it is that our AIs are able to know information, reason about that information, and draw conclusions, all in our look at AI
and the principles behind it. We'll see you next time.
Heads up!
This summary and transcript were automatically generated using AI with the Free YouTube Transcript Summary Tool by LunaNotes.
Generate a summary for freeRelated Summaries
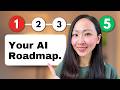
A Step-by-Step Roadmap to Mastering AI: From Beginner to Confident User
This video provides a comprehensive roadmap for anyone looking to start their AI journey, emphasizing the importance of understanding core concepts before diving into tools. It offers practical tips on building an AI learning system, developing critical thinking skills, and strategically selecting AI tools to enhance productivity.
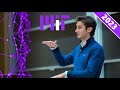
Understanding Introduction to Deep Learning: Foundations, Techniques, and Applications
Explore the exciting world of deep learning, its techniques, applications, and foundations covered in MIT's course.
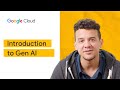
Understanding Generative AI: Concepts, Models, and Applications
Explore the fundamentals of generative AI, its models, and real-world applications in this comprehensive guide.
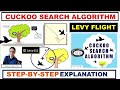
Understanding Cuckoo Search Algorithm: A Comprehensive Guide
Explore the Cuckoo Search Algorithm's principles, implementation steps, and its mathematical models.
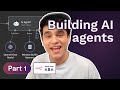
Building AI Agents with n8n: A Comprehensive Guide
Learn how to create AI agents using n8n for effective automation workflows in this detailed tutorial.
Most Viewed Summaries
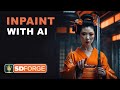
Mastering Inpainting with Stable Diffusion: Fix Mistakes and Enhance Your Images
Learn to fix mistakes and enhance images with Stable Diffusion's inpainting features effectively.
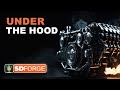
A Comprehensive Guide to Using Stable Diffusion Forge UI
Explore the Stable Diffusion Forge UI, customizable settings, models, and more to enhance your image generation experience.
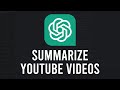
How to Use ChatGPT to Summarize YouTube Videos Efficiently
Learn how to summarize YouTube videos with ChatGPT in just a few simple steps.
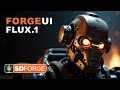
Ultimate Guide to Installing Forge UI and Flowing with Flux Models
Learn how to install Forge UI and explore various Flux models efficiently in this detailed guide.
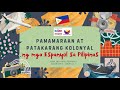
Pamaraan at Patakarang Kolonyal ng mga Espanyol sa Pilipinas
Tuklasin ang mga pamamaraan at patakarang kolonyal ng mga Espanyol sa Pilipinas at ang mga epekto nito sa mga Pilipino.