Introduction to Generative AI
Generative AI has emerged as a revolutionary branch of artificial intelligence that encompasses various sophisticated techniques capable of creating new content, including text, images, audio, and more. In this article, we will explore the fundamental concepts of generative AI, delve into its models and applications, and demonstrate how this technology is reshaping various industries. Whether you're a developer, a business owner, or simply curious about AI, this guide will provide the insights you need to understand generative AI better.
What Is Generative AI?
Generative AI refers to a subset of artificial intelligence technologies that enable machines to generate new content based on the patterns learned from existing data. This innovative technology has gained popularity for its ability to automate and enhance creative processes, making it a buzzword in the tech world. The foundation of generative AI lies in its ability to learn from data through various methodologies, allowing it to produce outputs that mimic human creativity.
Defining Key Terms
- Artificial Intelligence (AI): A branch of computer science focused on creating intelligent agents capable of autonomous reasoning, learning, and decision-making.
- Machine Learning (ML): A subfield of AI where algorithms learn from input data to make predictions or classifications without explicit programming.
- Deep Learning: An advanced subset of ML that utilizes artificial neural networks to process large volumes of data and learn intricate patterns.
Understanding these foundational concepts is crucial as we explore how generative AI fits into the broader context of AI technologies.
How Does Generative AI Work?
Generative AI models undergo training using vast datasets, which allows them to understand and replicate the underlying structures of the data. Here’s a breakdown of how generative AI works:
- Data Ingestion: Generative AI models are fed large amounts of both labeled and unlabeled data. This includes a wide range of content types such as text, images, and audio.
- Training: Through training, the models learn patterns, features, and relationships within the data. This occurs through methods such as supervised, unsupervised, or semi-supervised learning.
- Content Generation: Once trained, generative AI can create new content that appears similar to the training data. For example, a language model generates text responses based on the learned patterns of language.
Types of Generative Models
Generative models can be categorized into two main types:
- Generative Models: These models can create new data instances that resemble the training data. They focus on understanding the probability distribution of the data.
- Discriminative Models: These models classify or predict the labels of input data based on learned relationships. They do not generate new data; instead, they categorize existing instances.
To illustrate the difference, let’s consider an example of a generative model that can produce a new image of a dog based on learned features from numerous dog images. In contrast, a discriminative model would be used to classify an image as either ‘dog’ or ‘cat’ based on its features.
Exploring Generative AI Models
Generative AI encompasses various models, each specializing in different types of content generation. These models include:
1. Generative Language Models
Generative language models, like GPT-3, are designed to understand and produce human-like text. They learn language patterns by processing vast amounts of textual data, enabling them to complete sentences, answer questions, and even generate dialogue.
2. Text-to-Image Models
These models can create images from text descriptions. By using diffusion techniques, they synthesize images based on the textual input given by users. A notable example is DALL-E, which generates intricate and creative images from simple prompts.
3. Text-to-Video and Text-to-3D Models
Text-to-video models produce video content from textual scripts, while text-to-3D models create three-dimensional objects based on descriptions. These models represent a significant leap in AI's capability to visualize concepts in various dimensions.
4. Foundation Models
Foundation models are large AI models pre-trained on extensive datasets. They are designed to be adaptable for various downstream tasks, including sentiment analysis and object recognition. Google Cloud's Vertex AI offers Foundation models that cater to diverse applications in AI.
Practical Applications of Generative AI
Generative AI has a wide range of applications across different sectors. Here are some notable examples:
- Content Creation: Automated content generation saves time and resources for marketers, bloggers, and creatives by producing high-quality text, images, and videos.
- Coding Assistance: Tools like GitHub Copilot leverages generative AI to aid developers in code generation, debugging, and translation into different programming languages.
- Customer Support: AI-driven chatbots powered by generative models enhance user experiences and operational efficiency in customer service scenarios.
- Healthcare: AI can analyze medical data, assist with diagnostics, and even generate personalized treatment plans based on historical patient data.
Challenges and Considerations
Despite its incredible potential, generative AI also poses challenges:
- Data Quality: The effectiveness of generative models heavily depends on the quality and diversity of the training data. Poor or biased data can lead to inaccurate outputs.
- Hallucinations: Sometimes, AI models may produce nonsensical or misleading information. This phenomenon, known as 'hallucination,' requires careful evaluation of AI-generated content.
- Ethical Implications: The capacity of generative AI to produce human-like content raises ethical questions regarding copyright, misinformation, and the potential for misuse.
Conclusion
Generative AI has evolved into a powerful force in the realm of artificial intelligence, transforming how we create content and interact with technology. From text and images to innovative applications in various industries, this technology holds immense promise for the future. Understanding generative AI's fundamental concepts, applications, and challenges is essential for harnessing its full potential. As we continue to explore and develop AI technologies, the possibilities are endless, paving the way for a future where creativity, efficiency, and science coexist harmoniously.
[Music] hi and welcome to introduction to generative AI don't know what that is
then you're in the perfect place I'm Roger Martinez and I am a developer relations engineer at Google cloud and
it's my job to help developers learn to use Google cloud in this course I'll teach you four things how to Define
generative AI explain how generative AI Works describe generative AI model types describe generative AI applications but
let's not get swept away with all of that yet let's start by defining what generative AI is first generative AI has
become a buzzword but what is it generative AI is a type of artificial intelligence technology that can produce
various types of content including text imagery audio and synthetic data but what is artificial
intelligence since we are going to explore generative artificial intelligence let's provide a bit of
context two very common questions asked are what is artificial intelligence and what is the difference between Ai and
machine learning let's get into it so one way to think about it is that AI is a discipline like how physics is a
discipline of science AI is a branch of computer science that deals with the creation of intelligent agents and our
system systems that can reason learn and act autonomously are you with me so far
essentially AI has to do with the theory and methods to build machines that think and act like humans pretty simple right
now let's talk about machine learning machine learning is a subfield of AI it is a program or system that trains a
model from input data the trained model can make useful predictions from new never-before seen data drawn from the
same one used to train the model this means that machine learning gives the computer the ability to learn without
explicit programming so what do these machine learning models look like two of the
most common classes of machine learning models are unsupervised and supervised ml models the key difference between the
two is that with supervised models we have labels labeled data is data that comes with a tag like a name a type or a
number unlabeled data is data that comes with no
tag so what can you do with supervised and unsupervised models this graph is an example of the
sort of problem a supervised model might try to solve for example let's say you're the owner of a restaurant what
type of food do they serve let's say pizza or dumplings no let's say pizza I like
pizza anyway you have historical data of the bill amount and how much different people tipped based on the order type
pickup or delivery in supervised learning the model learns from past examples to predict future values here
the model uses a total bill amount data to predict the future tip amount based on whether an order was picked up or
delivered also people tip your delivery drivers they work really hard this is an example of the sort of problem that an
unsupervised model might try to solve here you want to look at tenure and income and then group or cluster
employees to see whether someone is on the fast trck nice work blue shirt unsupervised problems are all about
discovery about looking at the raw data and seeing if it naturally falls into groups this is a good start but let's go
a little deeper to show this difference graphically because understanding these Concepts is the foundation for your
understanding of generative AI in supervised learning testing data values X our input into the model the
model outputs a prediction and Compares it to the training data used to train the model if the predicted test data
values and actual training data values are far apart that is called error the model tries to reduce this error until
the predicted and actual values are closer together this is a classic optimization
problem so let's check in so far we've explored differences between artificial intelligence and machine learning and
supervised and unsupervised learning that's a good start but what's next let's briefly explore where deep
learning fits as a subset of machine learning methods and then I promise we'll start talking about
gen while machine learning is a broad field that encompasses many different techniques deep learning is a type of
machine learning that uses artificial neural networks allowing them to process more complex patterns than machine
learning artificial neural networks are inspired by the human brain pretty cool huh like your brain they are made up of
many interconnected nodes or neurons that can learn to perform tasks by processing data and making
predictions deep learning models typically have many layers of neurons which allows them to learn more complex
patterns than traditional machine learning models neural networks can use both
labeled and unlabeled data this is called semi-supervised learning in semi supervised learning a neural network is
trained on a small amount of labeled data and a large amount of unlabeled data the labeled data helps the neural
network to learn the basic concepts of the tasks while the unlabeled data helps the neural network to generalize to new
examples now we finally get to where generative AI fits into this AI discipline gen AI is a subset of deep
learning which means it uses artificial neural networks can process both labeled and unlabeled data using supervised
unsupervised and semi-supervised methods large language models are also a subset of deep learning see I told you
I'd bring it all back to gen good job me deep learning models or machine learning models in general can be divided into
two types generative and discriminative a discriminative model is a type of model that is used to classify
or predict labels for data points discriminative models are typically trained on the data set of labeled data
points and they learn the relationship between the features of the data points and the
labels once a discriminative model is trained it can be used to predict the label for new data
points a generative model generates new data instances based on a learned probability distribution of existing
data generative models generate new contents take this example here the discriminative model learns the
conditional probability distribution or the probability of Y our output given X our input that this is a dog and
classifies it as a dog and not a cat which is great because I'm allergic to cats the generative model learns The
Joint probability distribution or the probability of X and Y P of x y and predicts the conditional probability
that this is a dog and can then generate a picture of a dog good boy I'm going to name him Fred
to summarize generative models can generate new data instances and discriminative models discriminate
between different kinds of data instances one more quick example the top image shows a traditional machine
learning model which attempts to learn the relationship between the data and the label or what you want to predict
the bottom image shows a generative AI model which attempts to learn patterns on content so that it can generate new
content so what if someone challenges you to a game of is it gen or not I've got your
back this illustration shows a good way to distinguish between what is Gen and what is
not it is not gen when the output or why or label is a number or a class for example spam or not spam or a
probability it is Gen when the output is natural language like speech or text audio or an image like Fred from before
for example let's get a little mathy to really show the difference visualizing
this mathematically would look like this if you haven't seen this for a while the yal F ofx equation calculates the
dependent output of a process given different inputs the y stands for the model output the F embodies a function
used in the calculation or model and and the X represents the input or inputs used for the
formula as a reminder inputs are the data like comma separated value files text files audio files or image files
like Fred so the model output is a function of all the inputs if the Y is a number like predicted sales it is not
generative AI if Y is a sentence like Define sales it is generative as the question would elicit a text
response the response will be based on all the massive large data the model was already trained on so the traditional ml
supervised learning process takes training code and label data to build a model depending on the use case or
problem the model can give you a prediction classify something or cluster something now let's check out how much
more robust the generative AI process is in comparison the generative AI process can take
training code labeled data and unlabeled data of all data types and build a foundation model the foundation model
can then generate new content it can generate text code images audio video and more we've come a long way from
traditional programming to neural networks to generative models in traditional programming we
used to have to hardcode the rules for distinguishing a cat type animal legs four ears two fur yes
likes yarn catnip dislikes Fred in the wave of neural networks we could give the networks pictures of cats
and dogs and ask is this a cat and it would predict a cat or not a cat what's really cool is that in the generative
wave we as users can generate our own content whether it be text images audio video or more for example models like
Gemini Google's multimodal AI model or Lambda language model for dialogue applications ingest very very large data
from multiple sources across the internet and build Foundation language models we can use simply by asking a
question whether typing it into a prompt or verbally talking into the prompt itself so when you ask it what's a cat
it can give you everything it's learned about a cat now let's make things a little more
formal with an official definition what is generative AI gen is a type of artificial
intelligence that creates new content based on what it has learned from existing content the process of learning
from existing content is called training and results in the creation of a statistical
model when given a prompt gen uses a statistical model to predict what an expected response might be and this this
generates new content it learns the underlying structure of the data and can then generate new samples that are
similar to the data it was trained on like I mentioned earlier a generative language model can take what it has
learned from the examples it's been shown and create something entirely new based on that
information that's why we use the word generative but large language models which generate novel combinations of
texts in the form of natural sounding language are only one type of generative AI a generative image model takes an
image as input and can output text another image or video for example under the output text you can get visual
question and answering while under output image an image completion is generated and under output video
animation is generated a generative language model takes text as input and can output more
text an image audio or decisions for example under the output text question answering is generated and
under output image a video is generated I mentioned that generative language models learn about patterns in
language through training data check out this example based on things learned from its training data it offers
predictions of how to complete this sentence I'm making a sandwich for peanut butter
and jelly pretty simple right so given some text it can predict what comes next thus generative language models are
pattern matching systems they learn about patterns based on the data that you provide here is the same example
using Gemini which is trained on a massive amount of Text data and it's able to communicate and generate
humanlike texts in response to a wide range of prompts and questions see how detailed the response can
be here is another example that's just a little more complicated than peanut butter and jelly sandwiches the meaning
of life is and even with a more ambiguous question Gemini gives you a contextual
answer and then shows the highest probability response the power of generative AI comes from the use of
Transformers Transformers produced the 2018 revolution in natural language processing at a high level a Transformer
model consists of an encoder and a decoder the encoder encodes the input sequence and passes it to the decoder
which learns how to decode the representations for a relevant task sometimes Transformers run into
issues though hallucinations are words or phrases that are generated by the model that are often nonsensical or
grammatically incorrect see not great hallucinations can be caused by a number of factors like when the model is not
trained on enough data is trained on noisy or dirty data is not given enough context or is not given enough
constraints hallucinations can be a problem for Transformers because they can make the output text difficult to
understand they can also make the model more likely to generate incorrect or misleading information so put simply
hallucinations are bad let's pivot slightly and talk about prompts a prompt is a short piece of text that is given
to a large language model or llm as input and it can be used to control the output of the model in a variety of ways
prompt design is the process of creating a prompt that will generate desired output from an
llm like I mentioned earlier generative AI depends a lot on the training data that you have fed into it it analyzes
the patterns and structures of the input data and thus learns both with access to a browser
based prompt you the user can generate your own content so let's talk a little bit about
the model types available to us when text is our input and how they can be helpful in solving problems like never
being able to understand my friends when they talk about soccer the first is
texttext texttext models take a natural language input and produce text output these models are trained to learn the
mapping between a pair of text for example translating from one language to others next we have text to image text
to image models are trained on a large set of images each caption with a short text
description diffusion is one method used to achieve this there's also text to video and text to
3D textto video models aim to generate a video representation from text input the input text can be anything from a single
sentence to a full script and the output is a video that corresponds to the input text similarly texted 3D models generate
three-dimensional objects that correspond to a user's text description for use in games or other 3D
worlds and finally there's text to task text to task models are trained to perform a defined task or action based
on text input this task can be a wide range of actions such as answering a question performing a search making a
prediction or taking some sort of action for example a textto tax model could be trained to navigate a web user interface
or make changes to a doc through a graphical user interface see with these models I can
actually understand what my friends are talking about when the game is on another model that's larger than
those I mentioned is a foundation model which is a large AI model pre-trained on a vast quantity of data designed to be
adapted or find tuned to a wide range of Downstream tasks such as sentiment analysis image captioning and object
recognition Foundation models have the potential to revolutionize many Industries including healthare finance
and customer service they can even be used to detect fraud and provide personalized customer
support if you're looking for foundation models vertex AI offers a model Garden that includes Foundation models the
language Foundation models include chat text and code the vision Foundation models include stable diffusion which
have been shown to be effective at generating highquality images from text descriptions let's say you have a use
case where you need to gather sentiments about how your customers feel about your product or service you can use the
classification task sentiment analysis task model same for vision tasks if you need to perform occupancy
analytics there is a task specific model for your use case so those are some examples of
foundation models we can use but can gen help with code for your apps absolutely shown here are generative AI
applications you can see there's quite a lot let's look at an example of code generation shown in the second block
under the code at the top in this example I've input a code file conversion problem converting from from
python to Json I use Gemini and insert into the prompt box I have a pandas data frame
with two columns one with a file name and one with the hour in which it is generated I'm trying to convert it into
a Json file in the format shown on screen Gemini Returns the steps I need to do this and here my output is an a
Json format pretty cool huh well get ready it gets even better I happen to be using Google's free browser based
jupyter notebook and can simply export the python code to Google's cab so to summarize Gemini code generation can
help you debug your lines of source code explain your code to you line by line craft SQL queries for your database
translate code from one language to another and generate documentation and tutorials for source code I'm going to
tell you about three other ways Google Cloud can help you get more out of generative AI the first is vertex AI
Studio vertex AI Studio lets you quickly explore and customize generative AI
models that you can leverage in your applications on Google Cloud vertex AI Studio helps developers create and
deploy generative AI models by providing a variety of tools and resources that make it easy to get
started for example there is a library of pre-trained models tool for fine-tuning models tool for deploying
models to production and Community forum for developers to share ideas and collaborate next we have vertex AI which
is particularly helpful for all of you who don't have much coding experience you can build generative AI search and
conversations for customers and employees with vertex AI agent Builder formerly vertex AI search and
conversation build with little or no coding and no prior machine learning experience vertex AI can help you create
your own chat Bots digital assistants custom search engines knowledge bases training applications and
more lastly there is Gemini a multimodal AI model unlike traditional language models it's not limited to understanding
text alone it can analyze images understand the nuances of audio and even interpret programming code this allows
Gemini to perform complex tasks that were previously impossible for AI due to its Advanced Arch Ure Gemini
is incredibly adaptable and scalable making it suitable for diverse applications model Garden is
continuously updated to include new models and now you know absolutely everything about generative AI okay
maybe you don't know everything but you definitely know the basics thank you for watching our course and make sure to
check out our other videos if you want to learn more about how you can use AI [Music]
Heads up!
This summary and transcript were automatically generated using AI with the Free YouTube Transcript Summary Tool by LunaNotes.
Generate a summary for freeRelated Summaries
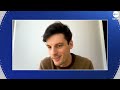
The Impact of Generative AI on Creative Industries and the Need for Protection
Explore the effects of generative AI on creative communities and discover ways to protect artists' work in a rapidly changing digital landscape.
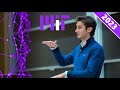
Understanding Introduction to Deep Learning: Foundations, Techniques, and Applications
Explore the exciting world of deep learning, its techniques, applications, and foundations covered in MIT's course.
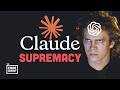
The Revolutionary Impact of Claude AI: A Game-Changer for Software Engineering
Explore how Claude AI surpasses GPT-4 and revolutionary features that redefine productivity.
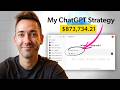
Unlocking Business Growth: Mastering AI Strategies for 2025
In this comprehensive video, discover how to effectively leverage AI, particularly ChatGPT, to enhance your business operations and marketing strategies. Learn about common pitfalls in AI content creation, the importance of authenticity, and actionable prompts to generate engaging content that resonates with your audience.
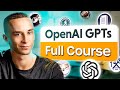
Unlocking the GPT Store: A Beginner's Guide to Creating AI Agents and Making Money
In this comprehensive guide, Liam Otley introduces the newly launched GPT Store, drawing parallels to the early days of the App Store. He shares essential skills and strategies for creating valuable GPTs, emphasizing the importance of unique offerings and effective marketing to stand out in a competitive landscape.
Most Viewed Summaries
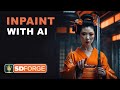
Mastering Inpainting with Stable Diffusion: Fix Mistakes and Enhance Your Images
Learn to fix mistakes and enhance images with Stable Diffusion's inpainting features effectively.
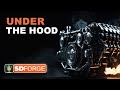
A Comprehensive Guide to Using Stable Diffusion Forge UI
Explore the Stable Diffusion Forge UI, customizable settings, models, and more to enhance your image generation experience.
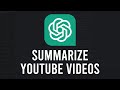
How to Use ChatGPT to Summarize YouTube Videos Efficiently
Learn how to summarize YouTube videos with ChatGPT in just a few simple steps.
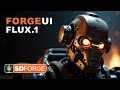
Ultimate Guide to Installing Forge UI and Flowing with Flux Models
Learn how to install Forge UI and explore various Flux models efficiently in this detailed guide.
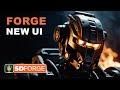
How to Install and Configure Forge: A New Stable Diffusion Web UI
Learn to install and configure the new Forge web UI for Stable Diffusion, with tips on models and settings.