Introduction
In today's competitive landscape, leveraging data is crucial for Human Resources (HR) departments to make informed decisions. This article provides a comprehensive overview of the data science framework applied specifically to HR analytics. We will explore an iterative process that enables HR professionals to define problems, collect data, build models, evaluate results, and ultimately make data-driven decisions.
Importance of HR Analytics
HR analytics, also known as people analytics, involves analyzing data related to HR metrics to support strategic decision-making. By utilizing a structured data science framework, HR professionals can improve recruitment processes, enhance employee retention strategies, and optimize workforce planning.
The Data Science Framework
The data science framework for HR analytics consists of several key steps: defining the goal, collecting data, building a model, evaluating that model, and presenting the results.
Step 1: Defining the HR Problem
The first step in the data science framework is to clearly define the HR problem you want to analyze. A well-defined problem statement includes the following components:
- What: Identify the specific HR issue you're focusing on (e.g., employee attrition).
- Why: Explain why this issue is significant (e.g., high attrition rates can impact productivity).
- Desired Outcome: Outline what you want to achieve (e.g., reducing attrition by 10% within six months).
Defining the problem is crucial as it informs the subsequent steps, including data collection and model selection.
Step 2: Collecting and Managing Data
Once the problem is defined, the next step involves gathering relevant data. Key considerations include:
- Sources of Data: Most organizations maintain a Human Resource Management System (HRMS), which serves as a valuable data source. However, data may also need to be collected from marketing, finance, and operations departments.
- Data Types: Understand the difference between structured and unstructured data. Ensure that your data analysis accounts for missing data and the quality of the data collected.
- Data Storage: Organize the data effectively, typically using spreadsheets or databases. This will facilitate further analysis.
Step 3: Building the HR Model
With your data in order, it's time to build your analytical model. The model should include:
- Dependent Variables: These are the primary variables you wish to analyze (e.g., employee attrition rates).
- Independent Variables: These are factors that may influence the dependent variables (e.g., employee satisfaction, salary levels).
During this phase, it’s vital to ensure that your data is trustworthy. Implement checks to validate the data's integrity since reliable data ensures accurate analysis and outcomes.
Step 4: Evaluating the Model
Once you build your model, the next step is to evaluate its effectiveness. This involves:
- Critiquing Metrics: Determine if your chosen metrics are suitable for analyzing the problem. Some metrics may have a closer relationship to the problem than others.
- Adjustments: Based on your evaluation, consider adjusting your metrics for better alignment with the defined goal.
Taking the example of measuring recruitment effectiveness, different metrics such as the number of applications and quality of hires can provide insights.
Step 5: Presenting the Results
The final step in the framework is to present the results of your analysis. This entails:
- Summarizing Findings: Clearly articulate the insights gained from the data analysis. Explain how these insights can inform HR strategies.
- Decision-Making: Present actionable recommendations based on your findings to stakeholders.
Conclusion
Understanding and applying the data science framework in HR analytics is a transformative process for HR professionals. By defining specific problems, effectively managing data, building robust models, and critically evaluating results, HR departments can make informed, data-driven decisions that enhance overall organizational performance. By fostering a culture of analytics within HR, organizations can better address workforce challenges and drive improvements in employee satisfaction and retention.
As we continue to navigate through the complexities of HR analytics, following a structured framework is essential for maximizing the impact of data-driven insights on HR strategies.
[Music] [Music] dear participant in first two session we
have learned learn the basics of the HR analytics so in first session if you remember we discussed the what are the
various names of the HR right how we should apply the basic concept related to the HR right so that is the basic
understanding that we had developed related to the HR analytics in first session in the second session we had
developed the basic understanding about the data right how we should develop the use the good dat data in order to
analyze and make some result related to the data analytics so in addition to that in first two sessions in this
session we will learn a framework through which you you can analyze the HR data so this framework that you can say
data science framework so any kind of analytics in any area that you are planning to apply this analytics you can
use this framework right so in HR area if we are applying so steps are same in marketing if you are applying or you are
applying any other area the steps are same but in so the framework that we we we will understand in HR context right
so what are the steps so here I have written the steps all steps of that data science framework right so that is the
content so first step is to define the goal right so that we are keep discussing in our sess
if you have to start this analytics first thing that you have to do you have to define the goal right so as we this
is the course related to the HR analytics so that is why we have written HR problem so any HR problem that is
what you have to Define right second step is related to the definition of HR problem should
cover so whatever problem that you are defining so you have to clearly Define the all things related to
that HR problem so right that is what you have to do next step that you have to you have the moment you have defined
the problem after defining it you have to collect the data related to it and manage it right so manage it means data
analysis structure data un structure data missing data quality of data so these are the all issues that we had
already discussed disc in the second so uh second session so this is the second step of the data science framework right
third after collecting the data you have to build a model right so in detail we will discuss in depth how you can build
a model in the HR so basically it is a matrix right that is a matrix that we need to develop so in the second session
if you remember we had discussed the how to develop the Matrix and what are the criteria for a good HR Matrix right so
here you have to build a model so that Matrix that you have to develop through which you will analyze the data the
moment you have developed this model right then you have to evaluate and critic the model why it is important
because we should know all matrics that we are developing right we should ensure that they are able to solve the problems
because all Matrix that we develop related to our problem they all all are proxy nobody no Matrix will give you the
exact thing right but some Matrix are better than others because they give you the
closure they have proximity to the defined goal right so in detail we along with the example this thing also we will
discuss right next is the moment you have developed the model then you evaluate and critic the model right you
evaluate whatever what are the limitations what are the strength of that particular Matrix whether it is
able to capture the real phenomena or not whe whatever that uh data wants to communicate whether you are able to
understand or not through that Matrix right so these all things that you have to do in this particular step right so
in detail we will discuss it and then finally you have to present the results right related to that particular model
whichever model that you have developed if you feel yes this results are good right and giving you uh the better
understanding about the problem then you can deploy that particular model in your organization so
these are the various steps of the data science framework right so whenever you
are applying this HR problem so I always suggest you go through this uh these steps right if you will randomly you
will do before if you have not defined the problem and collecting the data and then presenting the result without
evaluating that particular model that you have developed or that metrix so you may commit many mistakes while doing
this process so I suggest all HR managers to follow this these steps and apply the HR R Analytics tool so let us
start with First Step defining the HR problem right so being very clear on this is very important that defining it
is very important because the specific choices we make further about data and tools is fundamentally dependent on this
right so I hope you can understand why it is important because two reasons are there first reason is there if you are
not clear about about your goal what exactly that you want to do it right then you will not be clear about the
data which data that you are supposed to collect and which tool that you are supposed to use right so that's why it
is important so to define a problem so how you can define a problem like at how many people are
leaving right so then you can tell this is the percentage of people who are leaving from this particular Department
this particular reason people are leaving so you can do in-depth analysis of the at and then you will understand
you have to collect the data related to the attrition how many people are leaving the organization similarly
selection right so what kind of people if you want to do the analysis of selected people so out of 10,000 people
let us assume you have selected uh 200 people now you want to do the analysis of the selected people that quality of
high right how good these people are so now you understand you have to collect the data related to the quality of fire
because you want to understand how good they are if you want to understand their demography then you will collect the
data related to the demography so if your problem statement is is to evaluate the quality of quality of
people whoever whoever have been selected so you will collect the data related to the quality of fire right and
if your uh problem statement to understand the
demography demographics of the selected people from where they are what is their age what is their expectation what they
like what they don't like if you want to understand these all things then you will collect the data related to the
demography so that is what I was saying in the first step it is very very important to define the problem clearly
if you are unable to define the problem clearly you will not be able to select which type of data that you are supposed
to collect and which tool that you need to apply to analyze that particular data so that is why it becomes very very
important to define the problem clearly right whether it is related to the any function so I already said to Define
this problem you can select a HR function right whether it is related to recruitment
selection training development performance compensation and then you can go in depth then you can go in depth
and understand what is the problem and then you can decide which data that you have to collect related to the
Recruitment and which tool that you are supposed to apply so in the first session if you remember we had discussed
the various types of the statistics so descriptive statistics diagnostic statistics predictive statistics and
prescriptive statistics so again which type of Statistics that you will select that the method to analyze the tool
related to which type of analytics uh that you will select which tool that you will select related to which type of
Analytics tool that you will select that also will depend on the goal right if your problem is related in term of
exploring something then you may uh select the tool related to the descriptive like for example if you want
to understand how many people are leaving right then here simple count that you can apply from which department
they are leaving which area they are living which gender is living more right so problem is to understand
that particular phenomena that attrition phenomena so simply that you can apply descriptive Analytics tool if your
problem is is there any relationship between age and people who are leaving in that case you can apply this uh
diagnostic so in that case you can plot a correlation graph right so if you want to understand the relationship between
work uh uh work experience and the people who are leaving from the organization right so if you want to
understand who is leaving more people who are having more experience or less experience who are leaving more in whom
uh there is a high level of atra rate early joining people or people who are having more experience so the moment you
define a problem in this way it clearly indicates that you need to select a uh you need to select a tool from a
diagnostic statistics right so here you need to you can build a plot right you can make a graph who is leaving more
experienced people or inexperienced people or people who are having a specific scale those people so which
type of people are leaving more so that is what you that is what so in the first step that what I'm I'm trying to make
you understand first thing Define your problem clearly and why it is important because of two reason first is what type
of data that you need to collect that is dependent on your definition of your problem and second which type of tool
that you will use to analyze the data that is also dependent right so if you are not able to Define this problem or
illc conceived it is not appropriate in that case you may not be able to achieve the desired goal which you you are
expecting to achieve it right so this is the first step that is why it is always important to Define your problem clearly
right whatever uh problem that you are dealing with so related to the HR because we are discussing to the HR so
you can take functions and then related to the any HR any fun HR related function define what is the problem is
and then think about the data and Tool it is dependent on that so if your problem is not defined clearly then you
may face this problem so this is the first step of the data science framework let us move to the second right so now
question comes what ah HR problem should cover so statement of what and statement of Y and statement of
desired outcome so these are the three things that a problem statement should cover right so statement of what so what
is the problem attrition is the problem why attrition is a problem right because people are less paid people are more
experienced culture right department supervisor so why that problem exist I hope you can
understand the Aton that we are talking about so why people are leaving so through the data that you have to
explain right and now what exactly that you want to do it right or what you are trying to achieve it so you you want to
achieve the percentage of people who are leaving right and you want to know in which department most of the people are
leaving right so that that is what you want in the example that you can take related to The Oar operations efficiency
so in how many days you are able to complete the recruitment what is the cost of recruitment per application
right that per application cost per candidate selected candidate cost right what is the cost so so that these are
the matrics that you can calculate and then you can defied whether HR operations were efficient or not but you
have clearly identified for efficiency these are the parameters that you are that you want to calculate right so that
is what you have to Define so basically it is asking about the Matrix that is what on through which you want to
analyze that particular phenomena so these all three things your problem statement should have in order to make a
good problem statement related to the HR analytics so statement of what statement of why and the Matrix which through
which you will analyze this particular phenomena if you have these three things you will not face any problem so I have
given you the example of HR efficiency so I have taken the example of this recruitment so in the
recruitment uh what is the problem number of applications are less or more related to why it is less because of the
advertisement like so now you can uh calculate how much you have invested per application so let us assume you receive
10,000 so what is the amount and the total amount that you can divide by the 10,000 the total amount that you have
invested for the job advertisement right so that is how you can calculate per application cost that you have invested
so so that Matrix that you have already with you through this you want to describe this particular problem so
these are the three things that you should have when you are defining the HR problem so I hope you would have
understood next is collect the data and manage the data right so from where you will collect the data most of the
organizations are having HRS so this could be the good source of data right
sometime when you will develop the Matrix that time this HMS may not provide the complete
data which is required to analyze the data right which is required to analyze the data that may not be the may not
provide you the complete data because some data is available in marketing department some data is available in
finance Department some data is available in uh operations department so sometime this hrms may not provide you
the complete information right then what do you have to do you have already developed the
Matrix then think what could be the data source for the remaining data so this is the first step so you have checked your
hrms and what which data is not available think about that and then uh you can collect that data also so so the
moment you have collected the required data from various sources then what you can do then you can Define what are the
dependent variable and independent variable right so dependent variable is interest variable that is what I would
say so for example this work experience right if you want to do study related to the work experience right and
second thing salary right uh dissatisfaction so such kind of
variables that may come under the dependent variable so simple I would say which which variable is your
interest variable second is independent variable what is the reason why more experienced people are applying to your
organization right so work experience is your study variable dependent variable interest variable why more work
experience people are applying that is what you want to know so whatever is the reason you can say that because of
employer branding because of the salary that you uh provide the kind of culture that you have so these
culture salary right location these all things will come will come under the
independent variable because they because of this they more experienced people are
applying to your organization right so that is the that is how you have to Define after the collecting data how you
will decide this dependent variable which one is a dependent variable you can analyze what you can
analyze uh the your problem statement so in a problem statement what is your interest what exactly that you want to
know so from there you can drive or you can reach to the dependent variable and then from there whatever data is
available what could be the uh possible cause of that particular dependent variable why it is happening so through
the independent variable that you can do so when you are trying to establish some relationship between independent and
dependent variable you should have the logic behind it right so that lamp model if you remember in the first session
that is what we had discussed so lamp model so without any logic please do not consider all variables as an independent
variable right so you should have the logic uh this thing may lead to this particular thing right if that is not
there in that case you need to think about it right you need to think so all independent variable that you have to
identify through the uh logic right if logic is not there in that case you need to be worry about it without logic do
not select the independent variable so there has to be some logic then only you apply or you use that variable as a
independent variable right so the moment you have defined this variable independent variable dependent variable
whatever data was not there that also you have collected in a Excel format so now you need to perform three things
right extract transform and load extract transform and load Cod right so data
that you have extracted if that data is not as per the requirement right requirement for what
for the analysis right for example you are needed mean mean value right but mean value is not there right then what
you can do you can calculate the mean value and transform that particular data right so and then you can load on a
software through which you have to analyze the data so software that data visualization tool that we will learn in
this course pivot table through Excel powerbi and Tableau these are the three tools that we are going to learn so
before analyzing the data through these uh these three tools we need to uh transform that particular data and then
you have then we have to Lo load so these three terms that you can remember extract transform and load so whichever
data is not available then you have to extract if it is not up to the mark it means it is not uh in a same format in
which we require to analyze the data then we need to transform and then we need to load so these things that we
will learn when we will use the software in this course right so I hope you would have understood and second thing last
and uh not last I would say most important thing related to this data that we need to understand the
trustworthy if data is not trustworthy data should be trustworthy if it is not trustworthy otherwise garbage in and
garbage out whatever you will put you will get out right and you will not be able to make the meaningful results so
that's why you need to be very very careful about your data right you should check the trustworthy of n of all
trustworthiness of that particular data also so I hope you would have understood how to collect the data and manage the
data next build the model right so that model that I was talking about so that Matrix that you had decided right
to Matrix to describe the problem to understand the problem to find out the solution
right so now you have to decide the input data so if you remember that dependent variable independ
variable right is descriptive so all data that you have condu collected in Excel format so that will consider as a
input data so the moment you have identified this input data now you need to process this
particular step so now in a proc process step that you already know four type of statist uh analytic analytics that we
had discussed right so descriptive diagnostic predictive and Pres scriptive so in this step after finalizing the
input data you need to apply this particular analytical tool to analyze that data right and then you will get
the output so if this data is trustworthy your output also will be trustworthy if data is not trustworthy
then your output also cannot be trusted and you cannot take the decision base on this right so that is how you need to
build the model you need to input right and you need to check the output and you can apply so this is this is in
three format like input process and output so input data that you have already
identified based on your problem statement you have already finalized the tool so after finalizing the input data
now you need to apply the analytical tool it could be descriptive diagnostic predictive prescriptive and then you
will get the statistical outcome of it right then you will get a statistical outcome of it
right next so whatever model that you have developed right now you have to evaluate and critic the model so in this
step what exactly that you are supposed to do in this step you have to evaluate so whatever variable that you have
collected right why it is you have collected how close it is to the problem that is what you need to understand
right because every Matrix that you through which you collect the data that doesn't give you the exact that doesn't
describe the exact problem of the related to your goal right but each Matrix that you are collecting it should
have the higher level of the proximity to describe that particular problem or to ident if y the solution so if that
proximity Is Not to the highest level right for example you are having two Matrix right
both Matrix are giving describing the problem but one is close and another one is not that close right so for example
that I talk about the effectiveness of uh effectiveness of the Recruitment and selection activity right so two
numbers are there number of application number of application that is the one of the
Matrix and second one is quality of higher
right so both on the basis of both matrics you can establish the effectiveness of the recruitment right
so one Effectiveness that you can say number of application that has increased as compared to the last year and based
on that you can say that whether this recruitment was effective or not second that quality of people who have applied
to the available position based on that also you can say uh whether the recruitment was effective or not but
what do we want we want quality of people or number of application whether they are appropriate or not so it is
obvious we want quality of people right both so in this case you can see this quality of higher is having more
proximity to the effectiveness of recruitment than the application number of application although both uh matrixes
are very very important to understand the effectiveness of the recruitment but as far as my concern this quality of
high is more appropriate than the number of application right so so that is why I'm saying so you might be having
multiple matrices related uh to one particular problem so which one you have to select which one is more uh closer to
the problem right so that is how you have to evaluate and critic that particular model so that is how you have
to critic that particular problem particular model right and after CR after this then you have to present the
result whatever results that you are getting it right and if you find these results are suitable and then you can
deploy that particular model right so thank you I hope you would have learned the data science framework in this
session thank you [Music] a
[Music]
Heads up!
This summary and transcript were automatically generated using AI with the Free YouTube Transcript Summary Tool by LunaNotes.
Generate a summary for freeRelated Summaries
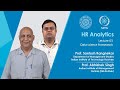
Mastering HR Analytics: A Comprehensive Guide to Data Science Frameworks
Unlock the potential of HR analytics with our guide to data science frameworks and methods for effective decision-making.
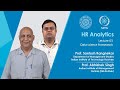
An In-Depth Guide to HR Analytics: Applying the Data Science Framework
Learn how to effectively apply HR analytics using a structured data science framework for optimal results.
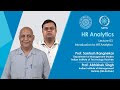
Understanding HR Analytics: A Comprehensive Guide
Explore HR analytics, its types, tools, and how HR managers can leverage it for informed decision-making.
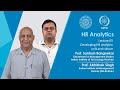
Developing HR Analytics Units: Essential Steps for Effective Data-Driven Decision Making
Explore key strategies to implement HR Analytics units for enhanced decision-making in organizations.
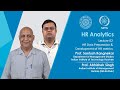
Comprehensive Guide to HR Data Preparation in Analytics
Learn essential steps for preparing HR data for effective analysis and decision-making in this comprehensive guide.
Most Viewed Summaries
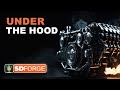
A Comprehensive Guide to Using Stable Diffusion Forge UI
Explore the Stable Diffusion Forge UI, customizable settings, models, and more to enhance your image generation experience.
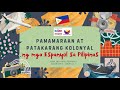
Pamaraan at Patakarang Kolonyal ng mga Espanyol sa Pilipinas
Tuklasin ang mga pamamaraan at patakarang kolonyal ng mga Espanyol sa Pilipinas at ang mga epekto nito sa mga Pilipino.
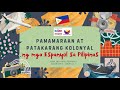
Pamamaraan at Patakarang Kolonyal ng mga Espanyol sa Pilipinas
Tuklasin ang mga pamamaraan at patakaran ng mga Espanyol sa Pilipinas, at ang epekto nito sa mga Pilipino.
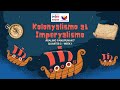
Kolonyalismo at Imperyalismo: Ang Kasaysayan ng Pagsakop sa Pilipinas
Tuklasin ang kasaysayan ng kolonyalismo at imperyalismo sa Pilipinas sa pamamagitan ni Ferdinand Magellan.
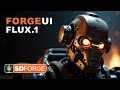
Ultimate Guide to Installing Forge UI and Flowing with Flux Models
Learn how to install Forge UI and explore various Flux models efficiently in this detailed guide.